As artificial intelligence (AI) plays a more significant role in decision-making, businesses and government institutions must ensure their machine learning models are transparent, trustworthy, and accountable.
However, many organizations struggle to measure explainability's return on investment (ROI). While transparency and fairness are clear benefits, leaders must understand how explainability can boost model performance, lower costs, and reduce risks.
This blog will cover:
- What explainability in AI means and why it matters.
- How explainability works and its role in AI governance.
- The business benefits of explainability.
- Challenges organizations face in AI explainability.
- How Fiddler’s explainability enhances model performance and optimizes ROI.
What is Explainability in AI and Why Does it Matter?
Explainable AI (XAI) refers to AI systems that provide clear insights into how they make decisions. Unlike traditional “opaque” models, which generate outputs without clear justification, explainable AI allows businesses to trace, interpret, and validate predictions to ensure accuracy and fairness.
Some AI models, such as decision trees and linear regression, are inherently interpretable, whereas deep learning models require specialized explainability techniques to make their decisions understandable.
Explainability is essential for:
- Trust and Adoption: Users are more likely to rely on AI systems they can understand.
- Regulatory Compliance: Many industries require auditable AI models to meet legal and ethical standards.
- Ethical AI Deployment: Explainability helps identify and mitigate biases in automated decisions.
The Significance of Trust, Transparency, and Governance in AI
Explainability in machine learning and AI is essential for organizations operating in regulated industries where transparency, fairness, and accountability are critical. Without it, businesses and government institutions risk bias, compliance violations, and diminished trust in AI-driven decisions —especially when using complex models that lack inherent interpretability.
Regulatory frameworks such as the EU AI Act, GDPR, and the U.S. AI Bill of Rights mandate transparency in high-risk AI applications, ensuring ethical and responsible deployment. By prioritizing explainable AI, organizations can strengthen governance, reduce risks, and ensure compliance, ultimately driving broader adoption and responsible AI integration across their operations.
Key industries that rely on AI explainability include:
- Finance: AI plays a critical role in credit scoring, loan approvals, fraud detection, and risk assessment, but deep learning systems often create opaque models that are difficult to interpret. Explainability helps financial institutions ensure transparency, regulatory compliance, and customer trust.
- Healthcare: AI-driven tools assist in diagnoses, risk assessments, and treatment planning, but their complexity can make decision-making challenging. Explainability improves transparency and adoption of generative AI models and deep learning systems, reducing the risk of biased or inaccurate recommendations.
- Government Agencies: AI is widely used in national security, law enforcement, and public policy. Explainability ensures that AI-driven decisions in criminal justice, border security, and public services are fair, unbiased, and accountable, supporting responsible AI governance.
How Explainable AI Works
Explainability methods vary depending on whether they are model-specific (intrinsically interpretable, such as decision trees) or model-agnostic (applied post-hoc to complex models like neural networks). Understanding a model's behavior becomes increasingly critical as AI systems grow more advanced — particularly in natural language processing and predictive performance applications.
Core Techniques for Model Explainability:
- Feature Importance: Identifying the most influential factors in a model’s decisions helps stakeholders understand why a particular prediction was made and how each variable contributed to the outcome.
- Partial Dependence Plots (PDPs): Illustrate how changes in a specific feature impact predictions, revealing nonlinear relationships.
- SHAP (Shapley Additive Explanations): Assigns a quantifiable value to each feature’s contribution, offering a more nuanced view of a model’s behavior.
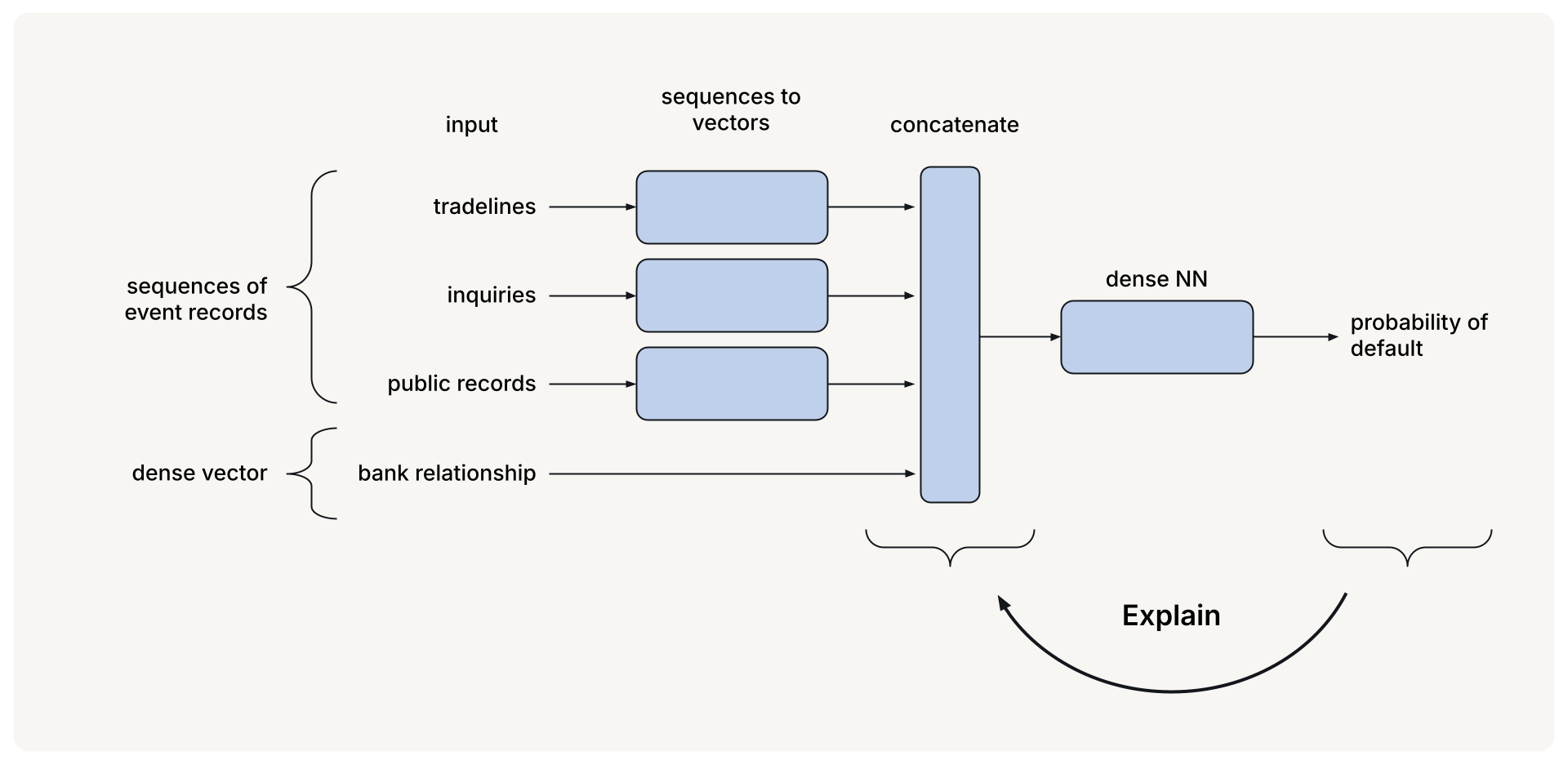
The Importance of Continuous Model Monitoring
AI models do not remain static — they evolve with new data, making continuous monitoring essential to maintaining accuracy, reliability, and fairness. Without ongoing assessment, models risk performance degradation, leading to unreliable predicted outcomes that can negatively impact decision-making.
Key factors contributing to model degradation include:
- Data Drift: Real-world data changes over time, creating differences between training data and testing data, which reduces model accuracy.
- Concept Drift: The relationship between input features and expected outputs shifts, making existing model parameters less effective in generating accurate predictions.
The Role of Explainability in Understanding Model Predictions
Explainability provides real-time insights into model predictions, helping organizations assess accuracy, fairness, and reliability in AI-driven decisions. Businesses can detect biases, refine strategies, and ensure regulatory compliance by understanding how models generate outputs.
Explainability also enables proactive model adjustments by identifying anomalies and monitoring prediction consistency. With a clear view of a model’s behavior, organizations can recalibrate decision-making processes, improve prediction accuracy, and maintain trust in AI systems.
Addressing Model Drift and Degradation
AI models degrade over time due to data drift, label shift, and performance decay, which can compromise accuracy. Explainability tools help pinpoint the root causes of these changes, allowing businesses to intervene before unreliable predictions affect outcomes.
Automated monitoring systems improve reliability by handling retraining and model adjustments, ensuring AI remains accurate, fair, and aligned with evolving business needs.
Benefits of AI Explainability
Explainable artificial intelligence (XAI) enables businesses and organizations to maximize the value of their AI models by improving trust, performance, and decision-making. Key benefits include:
1. Enhancing Trust and Reliability in AI-Powered Decisions
Explainability builds confidence in AI models by clarifying their decision-making processes to internal teams, regulators, and customers. With greater model transparency, stakeholders can trust that AI-driven outcomes are fair, unbiased, and accountable.
2. Boosting Performance While Optimizing ROI
Interpretable AI models improve efficiency and accuracy, reducing costly errors. By leveraging performance metrics, businesses can monitor AI effectiveness and make informed adjustments, ensuring models deliver high ROI while maintaining reliability.
3. Enabling More Informed Decision-Making
Explainability gives business leaders precise insights into AI recommendations, helping them understand how input data influences outcomes. Visualization tools allow decision-makers to assess trends and refine strategies based on AI-driven intelligence.
4. Understanding the Real-World Applicability of AI Outputs
AI models must align with industry-specific requirements and business contexts. Explainability helps ensure AI predictions remain relevant across the entire dataset, improving adaptability and applicability in real-world scenarios.
5. Mitigating Risks and Ensuring Fairness in AI Models
Bias in AI can lead to unfair or unethical outcomes. Explainability helps detect and mitigate biases, especially in tree-based models, ensuring that AI decisions remain fair, accountable, and compliant with ethical standards.
6. Regulatory Compliance
Many industries have strict regulations governing AI usage. Explainability supports auditable, explainable models, enabling organizations to meet legal requirements while demonstrating accountability in AI-driven decisions.
Challenges in AI Explainability and Model Evaluation
1. The Complexity of Model Interpretability
Deep learning models, such as neural networks, are often opaque systems, making it difficult to understand how they generate predictions. Unlike an interpretable model, which provides clear reasoning behind its outputs, neural networks require specialized explainability techniques to uncover the relationships between inputs and predicted outcomes.
2. Balancing Transparency with Performance
Achieving explainability can sometimes come at the cost of model efficiency and speed. Highly transparent models may be easier to interpret but not perform as well as more complex, opaque models. Organizations must find a strategic balance between interpretability and predictive accuracy to ensure AI models remain both understandable and effective.
3. Ethical and Regulatory Hurdles in AI Governance
Organizations must ensure fairness, data privacy, and accountability with evolving AI regulations to maintain compliance and public trust. Implementing governance frameworks aligned with industry standards is essential for ethical AI deployment.
How Fiddler’s Explainable AI Strengthens Your ML Model
Fiddler offers a comprehensive explainability platform that enhances transparency, security, and trust in AI-driven decisions. Businesses can use Fiddler to gain real-time insights, detect drift, and proactively adjust models, ensuring AI systems remain accurate and reliable.
Fiddler helps organizations align with industry regulations while improving model performance by providing transparent, accountable, and compliant AI decisions. Its built-in AI guardrails prevent biased, unfair, or unreliable predictions before deployment, keeping models trustworthy and high-performing. With Fiddler, businesses can confidently optimize AI models while mitigating risks and maximizing ROI.
Ready to build trust in your AI models? Dive into Fiddler Explainable AI to enhance model performance with transparency. Go beyond metrics — ensure your AI is understandable and resilient when issues arise.
Frequently Asked Questions about AI Explainability Tools
1. What is transparency and explainability in AI?
Transparency in AI means making model decisions clear and understandable, while explainability refers to the ability to describe how and why an AI model produces specific outcomes.
2. What is an example of explainability?
An example of explainability is SHAP (Shapley Additive Explanations), which quantifies each feature’s contribution to a model’s prediction, helping users understand its decision-making process.
3. What is explainability vs interpretability?
Interpretability focuses on understanding a model’s internal logic (e.g., decision trees), while explainability ensures that AI-generated outputs are clear to non-technical stakeholders.