We are thrilled to share major upgrades to the Fiddler AI Observability Platform to support Large Language Model Operations (LLMOps)! Effective monitoring is essential for maintaining the correctness, safety, scalability, and cost of LLM applications. Our team recently showcased the Fiddler LLM Enrichments Framework, which enables high-accuracy LLM monitoring.
How LLM Observability can Help Reduce AI Risks
According to a recent McKinsey & Company survey, the adoption of generative AI has increased significantly over the past year, with 65 percent of respondents indicating that their organizations regularly use generative AI (GenAI) in at least one business function. As the adoption of GenAI accelerates, enterprises must safeguard their company, employees, and customers from associated AI risks and challenges.
Consider the following real-life examples that highlight the risks posed by GenAI and LLM applications and how LLM Observability could have helped mitigate the risks:
Hallucinations in Legal Contexts
One of the most glaring issues with LLMs is their tendency to "hallucinate" — generating responses that appear plausible but are factually incorrect or entirely fabricated. A stark example of this occurred in New York, where a lawyer used ChatGPT to assist with legal research. ChatGPT provided six sources of information to support the lawyer's case. Unfortunately, it was later discovered that all six sources were entirely fabricated.
Jailbreak in Business Applications
Another example that underscores the necessity of LLM monitoring involves a chatbot deployed by a car dealership. A user manipulated the chatbot into stating that the purchase of a truck would only cost one dollar and claimed this response was legally binding. This situation, known as a "jailbreak," occurs when users exploit vulnerabilities in the LLM to generate unintended responses that negatively impact the company or customers.
Bias in GenAI Applications
Bias in GenAI applications is another significant concern, as it can lead to discriminatory outcomes that affect marginalized groups. An example of this is a chatbot that provided biased responses, discriminating against certain protected groups. This not only poses ethical and legal challenges but also undermines the trust of GenAI applications.
The Fiddler LLM Enrichments Framework for LLM Monitoring
LLMs operate on unstructured data like text, which is more nuanced than structured data. In addition, the accuracy of an LLM's output is highly context-dependent. What may be considered a good response in one context may be incorrect in another. As a result, monitoring the quality, correctness, and safety of outputs requires sophisticated techniques beyond simple accuracy metrics.

We’ve built the LLM Enrichments Framework to address this complexity. The LLM Enrichments Framework augments LLM inputs and outputs with scores (or "enrichments") for monitoring purposes.
The framework serves a wide range of scores for hallucination and safety metrics, such as faithfulness, answer relevance, toxicity, jailbreak, PII, and other LLM metrics, as seen on the diagram below. Whether it's a topic poorly covered by a chatbot's knowledge base or a vulnerability to specific prompt-injection attacks, the Fiddler LLM Enrichments Framework offers high accuracy LLM monitoring to enhance LLM application performance, correctness, and safety.
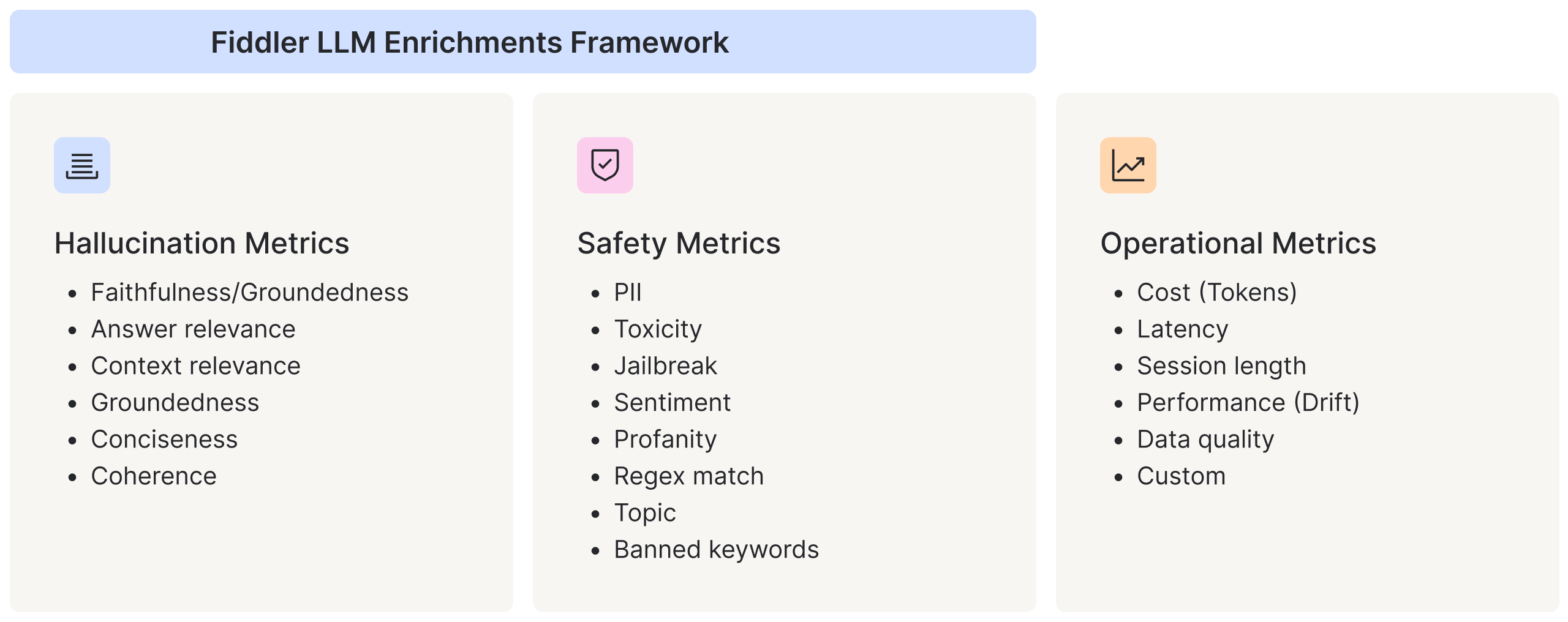
How the LLM Enrichments Framework Works
The Fiddler LLM Enrichments Framework generates enrichments to calculate prompts and responses and improve the quality of monitoring. Enrichments enhance user prompts and LLM responses with supplementary information for accurate scoring.
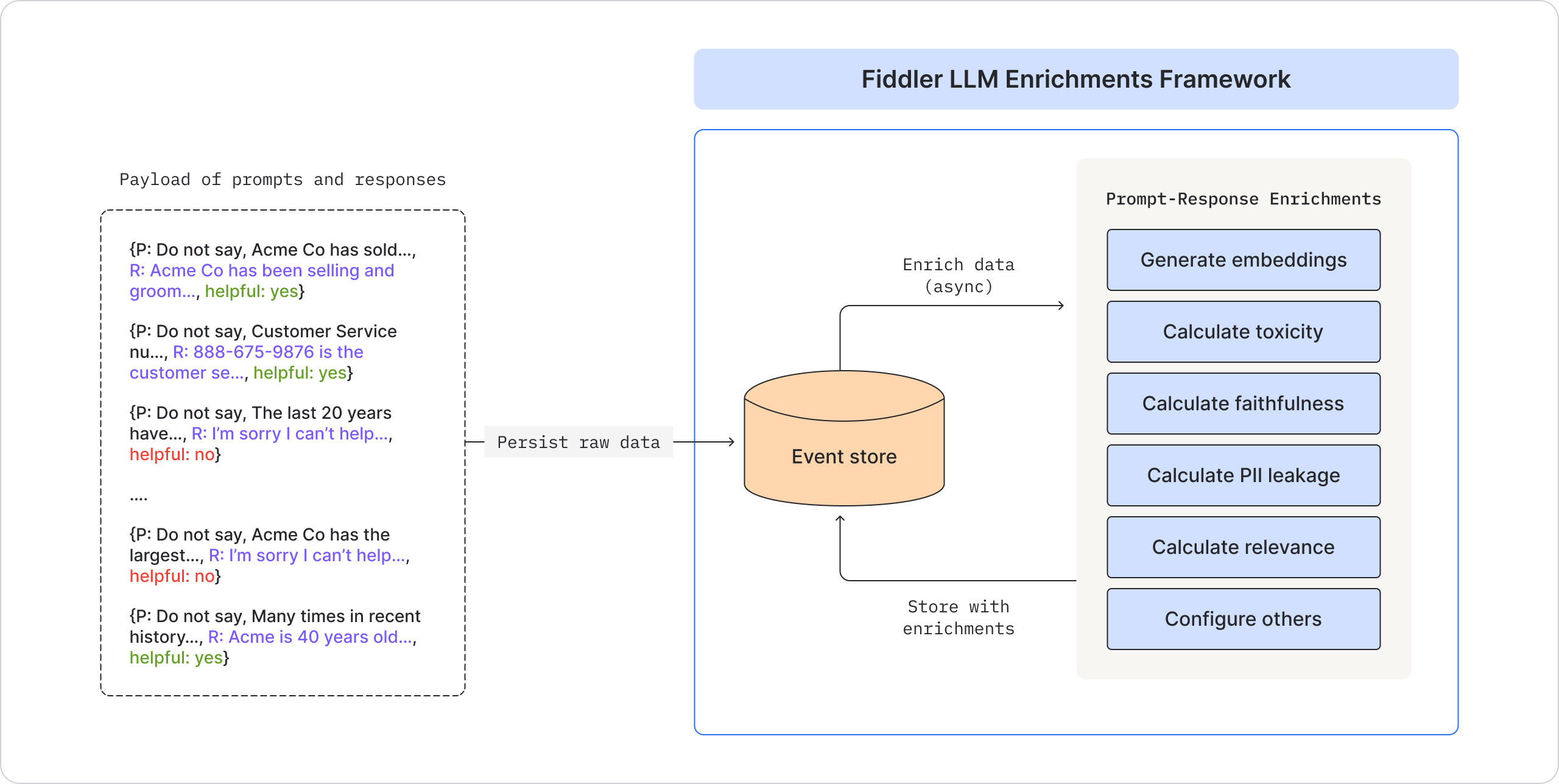
This process involves the following steps:
- Data Ingestion: Data, user prompts and LLM responses, are ingested into the Fiddler platform
- Data Scoring: Supplementary information is added to the data through specific calculations for the LLM metric being monitored, enhancing the quality and accuracy of the data
- LLM Metrics Monitoring: Enrichments use Fiddler Trust Models to understand the context behind the prompts and responses, providing an accurate score for LLM metrics monitoring in Fiddler
Fiddler Trust Models for Enterprise Scale LLM Monitoring
Behind the Fiddler LLM Enrichments Framework are the Fiddler Trust Models, our proprietary fine-tuned models, that quickly calculate scores for user prompts and LLM responses.
A common approach to score prompts and responses is using closed-source LLMs. However, this is a short-term solution that hinders enterprises from scaling their LLM application deployments in a cost-effective manner. Closed-source LLMs, with their hundreds of millions of parameters, are highly expressive and designed for general purposes, which increases the latency, and limits their effectiveness and efficiency in scoring specific metrics.
Unlike other LLMs, the Fiddler Trust Models are optimized for speed, safety, cost, and task-specific accuracy, delivering near real-time calculations. This efficiency helps enterprises scale their GenAI and LLM deployments across their organization effectively.

Key advantages of the Trust Models are:
- Fast: Obtain faster calculations to detect hallucinations, toxicity, PII leakage, prompt-injection attacks, and other metrics in near real-time
- Safe: Ensure data is secure and never leaves the premises, even in air gapped environments
- Scalable: Scale LLM applications as they get more user traffic
- Cost-effective: Maintain the costs to operate LLMs low
We are excited to share our latest platform upgrades to help enterprises scale their production GenAI applications. Watch the full webinar to learn more about Fiddler’s LLM Enrichments Framework.