We often consider the benefits of AI and machine learning, but what about the potential for harm? As more aspects of our lives have moved online due to the COVID-19 pandemic, the influence of AI systems is rapidly accelerating. It’s more important than ever to ensure that AI is used ethically and responsibly.
As part of Fiddler’s 3rd Annual Explainable AI Summit in October 2020, we brought together a diverse panel of experts in responsible AI. They shared what they’ve learned from working with some of the world’s largest businesses and governments to create and uphold standards for implementing AI systems. In this article, we’ll walk through some of the key points from our conversation; you can also watch the full-length video of our discussion here.
What is responsible AI?
“Responsible AI” refers to the responsible and ethical use of AI. That is, when we’re talking about responsible AI, we’re referring to decisions being made responsibly by humans involved in the design and implementation of AI systems. Ethics come into play because they are the guiding principles that help a company, or an entire society, decide on what it means to act for the greater good (which sometimes might mean not using AI to solve a certain problem, if the solution could cause harm).
Why it matters
Responsible AI is critical when it comes to systems making automated decisions that might have an impact on a person’s health, well-being, or access to resources and opportunities. We could extend this to include any impact on the environment and climate change as well. In 97 Things About Ethics Everyone in Data Science Should Know, panelist Bill Franks has written about potential pitfalls. One of the most thought-provoking challenges? Monitoring autonomous weapons.
Use cases for responsible AI can be found in all walks of life. Consider the idea of using AI systems in education for admission into a university, or for resume screening to connect job-seekers with recruiters, or in healthcare. Not all of these domains necessarily have representative data or ground-truth labels. This makes responsible AI even more important, since when you are generalizing based on a small slice of the population, it’s going to be hard to avoid bias and achieve equitable outcomes.
Implementing responsible AI
Implementing responsible AI is about ensuring that the behavior of your AI system is consistent with the requirements that you have defined. How can you succeed at this deceptively simple task? Here are some frameworks our panelists shared for putting responsible AI into practice.
5 Key Principles
- Reliability: How do model predictions change in different contexts? How generalizable is the model?
- Fairness: By using this model, are we making sure we aren’t creating or reinforcing bias?
- Interpretability: Do we know why the model made its predictions? Can we generate diagnostics that will offer transparency to a wide audience: data scientists, governments, and ordinary people affected by the model’s outputs?
- Privacy: Have we made sure that the data for our model respects user privacy and complies with all laws and regulations?
- Security: Is our model safe from attacks that might poison the data? Are we making sure the model doesn’t leak sensitive information?
Operational Procedures
It can also be useful to identify the steps that your organization can take to operationalize its principles around responsible AI. These might look like:
Step 1: Document the desired behavior of the AI systems and the larger product that they fit into (for example, a face-detection system for airports). What are the key performance indicators (KPIs) that will show whether the product is behaving as desired?
Step 2: Record the actual behavior while the system is working. A lot of the work here will involve translating the system’s decisions and the context in which those decisions were made into language that all stakeholders can understand (including end-users).
Step 3: Proactively look for what could go wrong. Organizations are used to taking a reactive approach to issues. But for responsible AI, where regulations are still emerging, it’s very important to actively identify all the potential areas of failure.
Step 4: Put an internal auditing and reporting process in place, and make sure that these reports can be accessed by all your stakeholders, including end-users and customers.
Our panelists emphasized that the final step, transparency, is critical. When an organization like the World Economic Fund implements ethical AI, they are reimagining regulation, working with governments and regulators to create new policies and frameworks.
Cultural Changes
Above all, responsible AI is only possible if you have a culture that supports it. As AI ethicist Merve Hickok explained, quoting Peter Drucker, “Culture eats strategy for breakfast.” The intention to have ethical AI needs buy-in across the company, from C-level executives to the developers implementing the models. We also need to have better cross-functional alignment, and give risk and compliance experts more tools that help them communicate with data scientists (and vice versa).
Implementing AI ethically is a challenge that businesses and governments will continue to grapple with, especially as the pace of AI implementation accelerates. Panelist Arnobio Morelix is writing a book, The Great Reboot, about shifts that will happen in a post-pandemic world. If we can use this moment to push for positive cultural changes and implement practical solutions, perhaps there will soon come a day when we don’t have to explain what “responsible AI” means — because this term will already be part of the mainstream.
This article was based on a conversation that brought together panelists from financial institutions, as part of Fiddler's 3rd annual Explainable AI Summit on October 21, 2020. You can view the recorded conversation here.
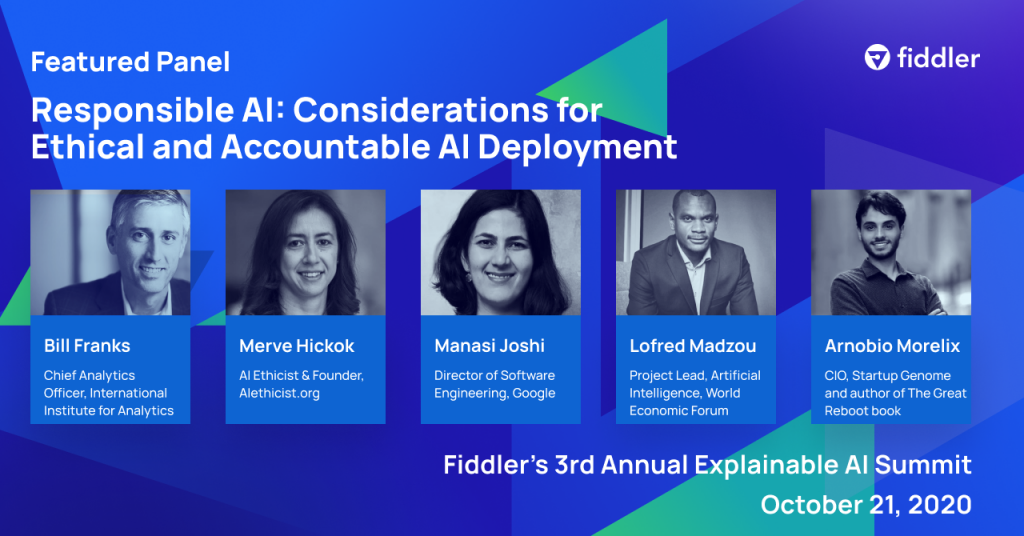
Panelists:
Arnobio Morelix, Research & Data Science Leader
Manasi Joshi, Director of Software Engineering, Google
Merve Hickok, AI Ethicist & Founder, AIethicist.org
Bill Franks, Chief Analytics Officer, International Institute for Analytics
Lofred Madzou, Project Lead, Artificial Intelligence, World Economic Forum
Moderated by Anusha Sethuraman, Head of Marketing, Fiddler