Ring out the old, ring in the new,
Ring, happy bells, across the snow:
The year is going, let him go;
Ring out the false, ring in the true.
Alfred Lord Tennyson, 1809 - 1892
As we stand on the brink of a technological transformation that will fundamentally alter the way we live, work, and relate to one another – I am reminded of this famous line from one of the Tennyson’s poems I used to recite as a young boy growing up.
Artificial Intelligence is set to dramatically change human lives – optimize jobs and activities, minimize risks, help us make effective decisions – however there are many questions that remain to be answered and many concerns that need to be addressed. It is a no-brainer that every enterprise whether public or private needs to be adopting AI today, at the risk of being obsolete and outgunned by the competition.
There are two main challenges that businesses face today with adopting AI
- Higher costs of building AI products.
- Increasing lack of trust in AI.
Operationalizing AI applications continues to be prohibitively time-consuming and expensive even for the most sophisticated companies. This is primarily because the tools that researchers and scientists use for building AI models are not scalable for real production systems. These systems need end-to-end AI platforms for everything from data preparation and labeling, to operationalization and monitoring. Additionally, the ROI ambiguity of AI within the enterprise makes them pursue a ‘golden use case’ thus holding back many from fully exploiting its potential. Existing enterprise AI platforms, especially those deployed on-premise, have poor UX, limited features and lack distributed computing. The only alternative for enterprises seems to be to either move to cloud offerings like AWS, Google Cloud or Azure ML, or start custom engineering projects.
Enterprises therefore are investing in significant R&D to build custom AI infrastructure that they need. The biggest tech companies have had a considerable head start here. First, they pioneered data collection, data engineering, and ML frameworks. Now they are building a new kind of proprietary infrastructure in-house e.g. FBLearner at Facebook, TFX at Google, Michelangelo at Uber, Notebook Data Platform at Netflix, Cortex at Twitter and BigHead at Airbnb. We call this kind of infrastructure, the ‘AI Engine’. This infrastructure manages compute loads, automates deployments for ML models, and provides tools for managing AI projects across the organization.
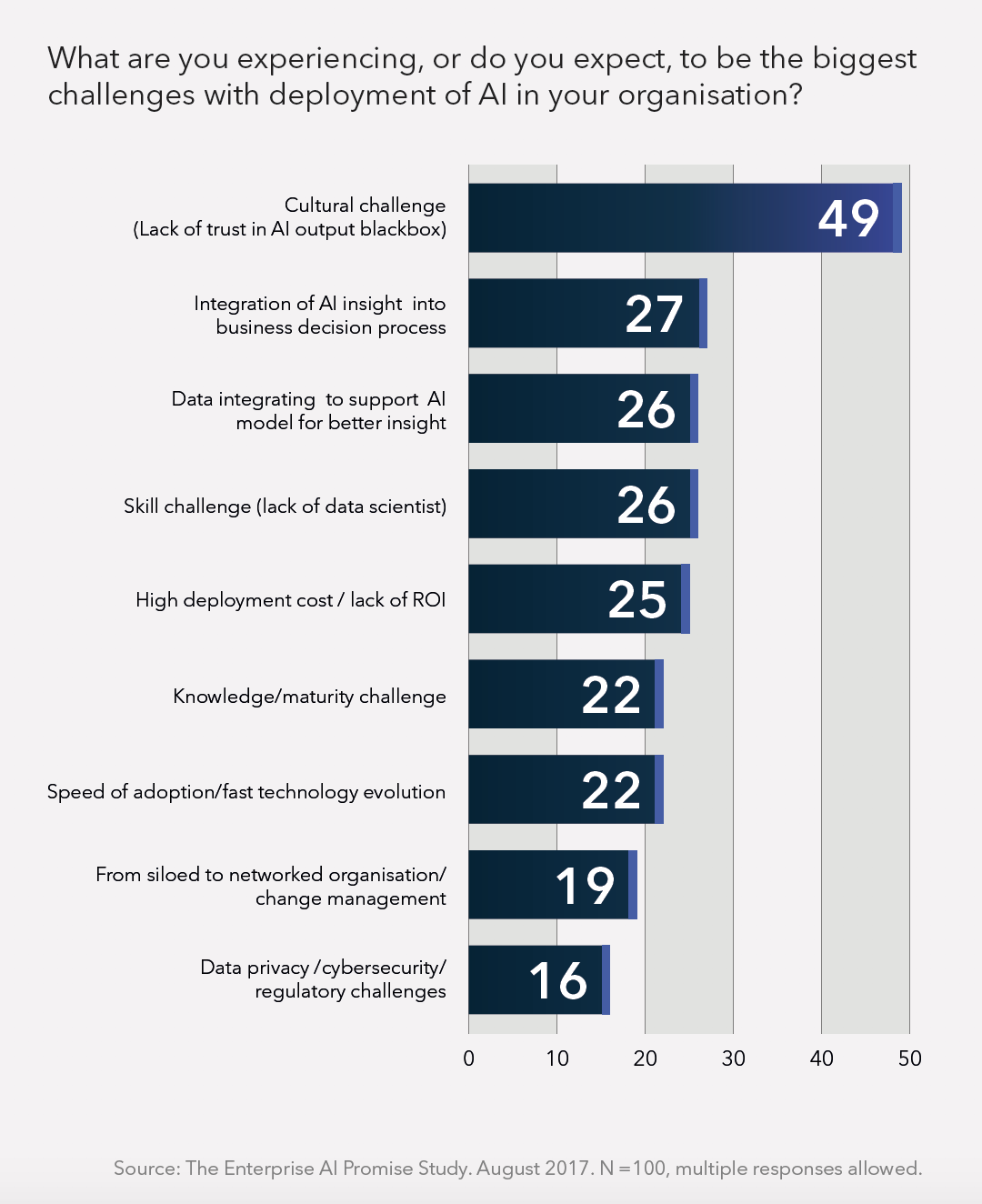
Trust in AI is a looming societal and technological problem.
One of the biggest questions people are concerned about: Is bias creeping into AI, or is it already present in the data that is fueling models? Fairness in AI poses a difficult and subjective question. Sometimes there can be a trade-off between accuracy and fairness of ML models. In sensitive applications such as healthcare or criminal justice, this trade-off is often undesirable as any increase in prediction error could have dangerous consequences. AI fairness is tricky because we cannot design a randomized experiment with race or gender. Instead, we need to understand and monitor data throughout the lifecycle of AI from generation, collection, sampling, feature engineering etc. It’s a good thing that as a society, we have become more sensitive about how we use data. There is also a clear consensus emerging that businesses need to be alert for the dangers of bias and its harmful effects on AI-powered decision-making.
To solve these problems, we have started Fiddler Labs to help enterprises adopt cutting edge AI by simplifying operationalization, removing the ambiguity around ROI, and crucially, by creating a culture of trust.
Converting data into intelligence has been our team’s multi-decade journey through companies like Facebook, Google, Twitter, Pinterest, Amazon, Lyft, PayPal and Microsoft. Over the years, we have seen many new tools, algorithms, and systems built, deployed and scaled in production. Our team has worked with business owners, data scientists, business analysts and devops to develop a deep understanding of the challenges they face day-to-day in converting data into intelligence and insight. These experiences led us to build a new kind of AI Platform that’s both more trustable and more efficient: The Explainable AI Engine.
You can contact us for more information or follow us @fiddlerlabs for updates. And if you are interested to help build the future of AI, join us!