Last week, President Biden issued an Executive Order on safe, secure, and trustworthy artificial intelligence. The executive order focuses on establishing safety and security standards, protecting privacy, advancing equity and civil rights, supporting consumers, workers, and innovation, and promoting U.S. leadership in AI globally.
The executive order highlighted 6 new standards (summary included at the end of this blog) for AI safety and security, such as mandating enterprises to share safety results of LLMs before launching into production, and establishing and applying rigorous AI safety standards, and protecting people from AI-enabled fraud and deception.
In light of the Executive Order, the Fiddler AI Observability platform emerges as a pivotal platform for enterprises. As more AI regulations are issued, protecting end-users against AI risks is a core concern amongst enterprises. We have talked to AI leaders and practitioners from enterprises across industries seeking expert advice on how to build an LLM strategy and execution plan, and partner with a full-stack AI Observability platform that spans from pre-production to production.
Based on their feedback, we are pleased to announce significant upgrades that expand the Fiddler AI Observability platform for LLMOps and help address concerns in AI safety, security, and trust.
Validate, Monitor, Analyze, and Improve LLMs
We have bolstered our AI Observability solution to provide enterprises with an end-to-end LLMOps workflow that enables AI teams to validate , monitor, analyze, and improve LLMs.
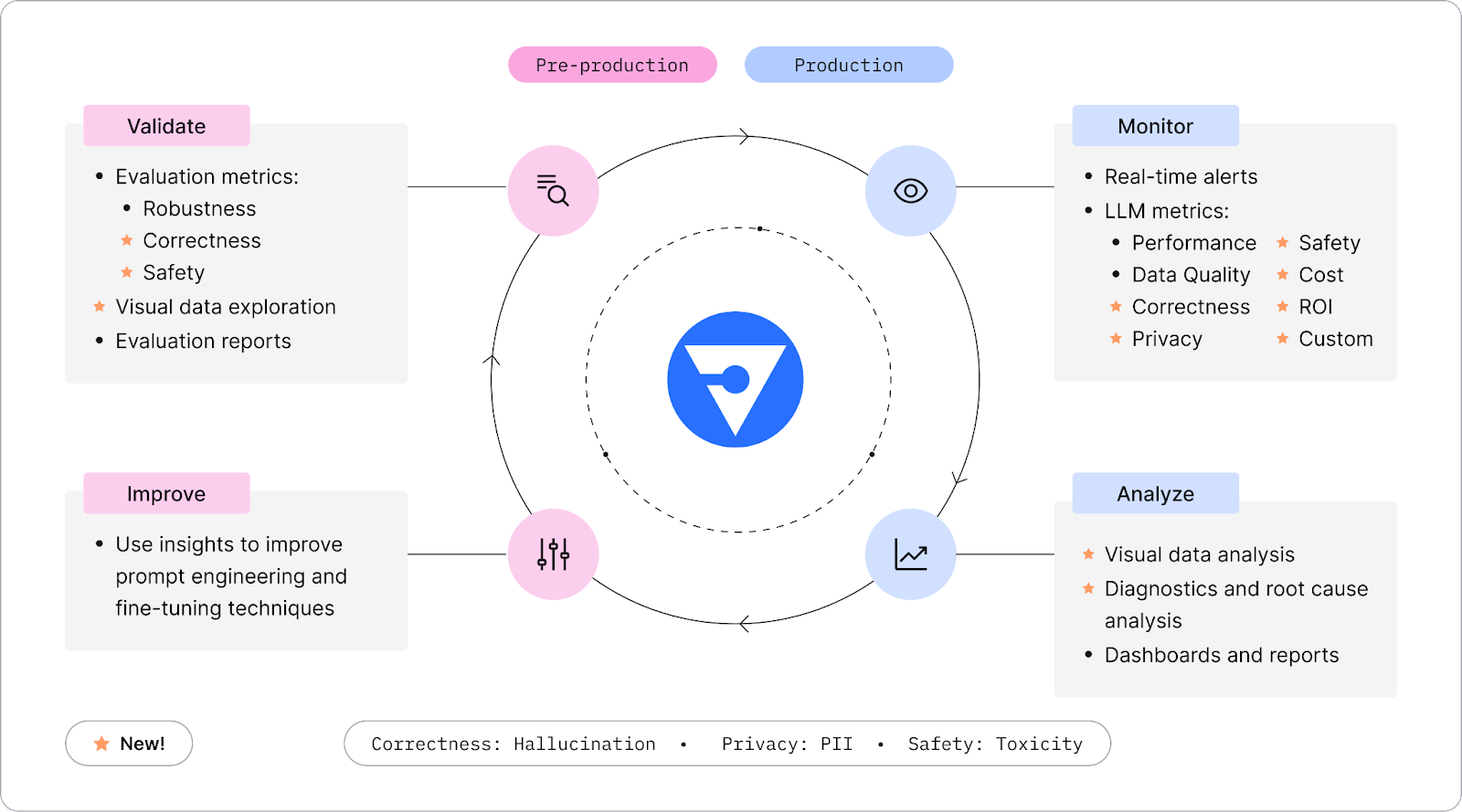
Pre-production: Validate Phase
LLM Evaluation and Unstructured Data Exploration
In conjunction with our core platform, Fiddler Auditor, the open-source library for red-teaming of LLMs, provides pre-production support for robustness, correctness, and safety.
Fiddler Auditor’s new capabilities to evaluate LLMs include:
- Model-Graded Evaluations: Lower operational costs by using a larger, more expensive model to automatically evaluate the more cost-effective model for robustness, correctness, and safety and save time from manual reviews
- Custom Transformations: Teams can evaluate LLMs against prompt injection attacks and identify LLM weaknesses to minimize deceptive and fraudulent actions that can harm users
- Safety Evaluation: Toxicity evaluation to assess potential harm and risks associated with the LLM’s responses.
- Support for Hugging Face datasets: Integrated support for Hugging Face datasets directly into the Fiddler Auditor.
Teams can also visually explore the prompts and responses, using the 3D UMAP in the Fiddler AI Observability platform, to understand unstructured data patterns and outliers.
Production: Monitor Stage
Monitoring LLMs for Safety, Correctness, Privacy, and Other LLM Metrics
In line with the Executive Order's focus on AI safety and privacy, the Fiddler AI Observability platform capabilities are key. The platform's ability to provide real-time model monitoring alerts on metrics like toxicity, hallucination, or personally identifiable information (PII) levels are industry leading.
The Fiddler AI Observability platform monitors metrics for the following categories:
Monitor Custom Metrics Unique to the Use Case
In addition to the out-of-the-box LLM metrics offered in our platform, customers are able to monitor and measure metrics unique to their use case using custom metrics. Customers can input their own distinct formula into Fiddler, allowing them to monitor and analyze these custom metrics as seamlessly as they would with standard, out-of-the-box metrics.
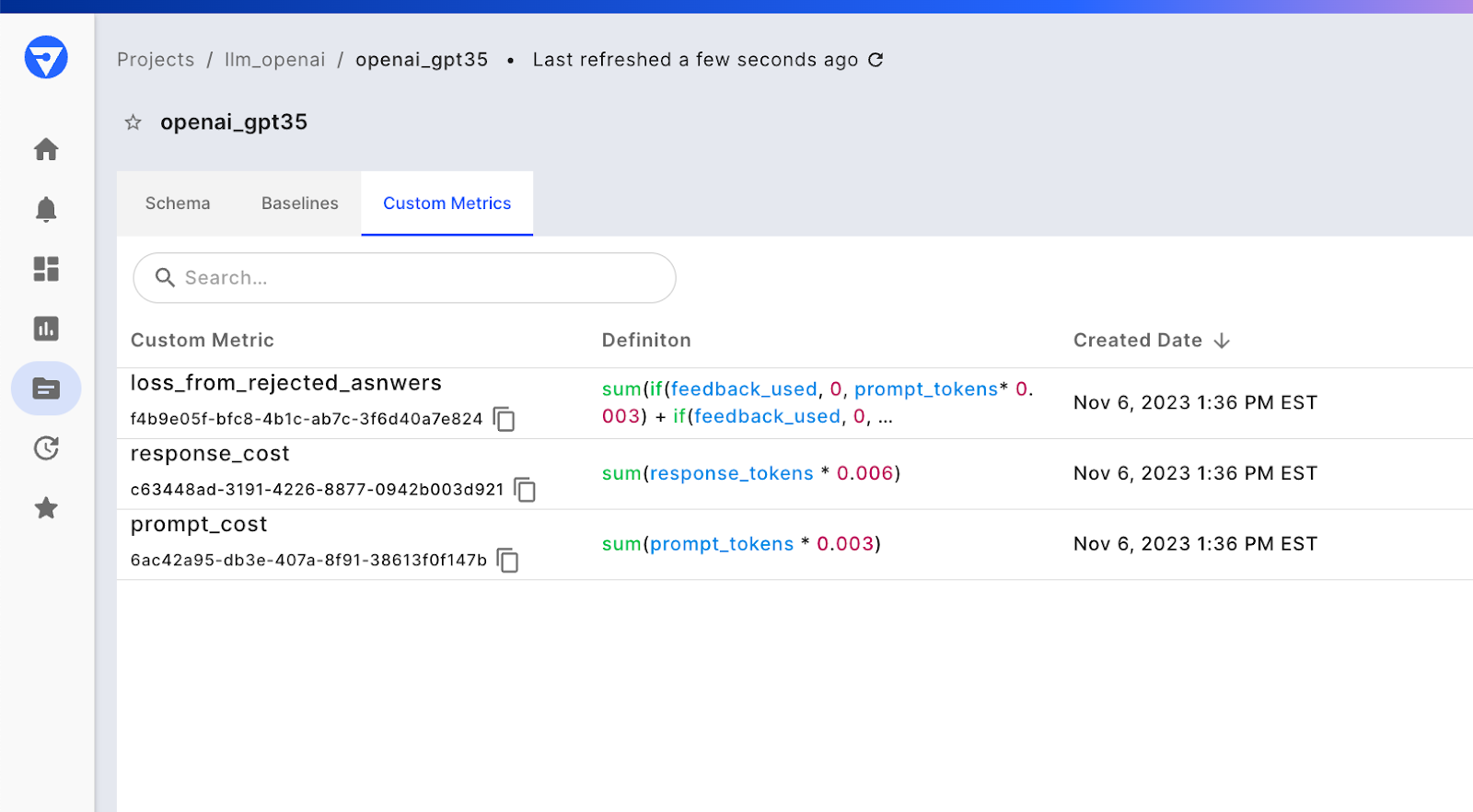
For instance, a customer who recently launched a chatbot wanted to monitor the associated cost. They created a custom response cost metric to track each OpenAI API call from the chatbot. And a separate custom metric to track the rejected responses generated by the chatbot — responses that end-users have deemed poor or unhelpful. By combining these two metrics in Fiddler into a single formula, the customer can calculate the true cost of using the chatbot. This approach allows them to gain a holistic understanding of the true financial impact and effectiveness of their chatbot.
Production: Analyze Stage
Analyze and Pinpoint Problems in Unstructured Data
The Fiddler 3D UMAP allows users to visualize unstructured data (text, image, and LLM embeddings), to discern patterns and trends in high-density clusters or outliers that are impacting the LLM’s responses, such as toxic interactions. Data Scientists and AI practitioners can overlay pre-production baseline data to compare how prompts and responses in production differ from this baseline. They can also gain contextual insights from human-in-the-loop feedback (👍👎) to gauge the helpfulness of LLM responses, and use that feedback to improve the LLM.
Customers can download the identified problematic prompts or responses from the 3D UMAP and use this dataset for prompt-engineering and fine-tuning.

Measure, Report, and Collaborate to Improve LLMs
Create intuitive dashboards and comprehensive reports to track metrics like PII, toxicity, and hallucination, fostering enhanced collaboration between technical teams and business stakeholders to refine and improve LLMs.
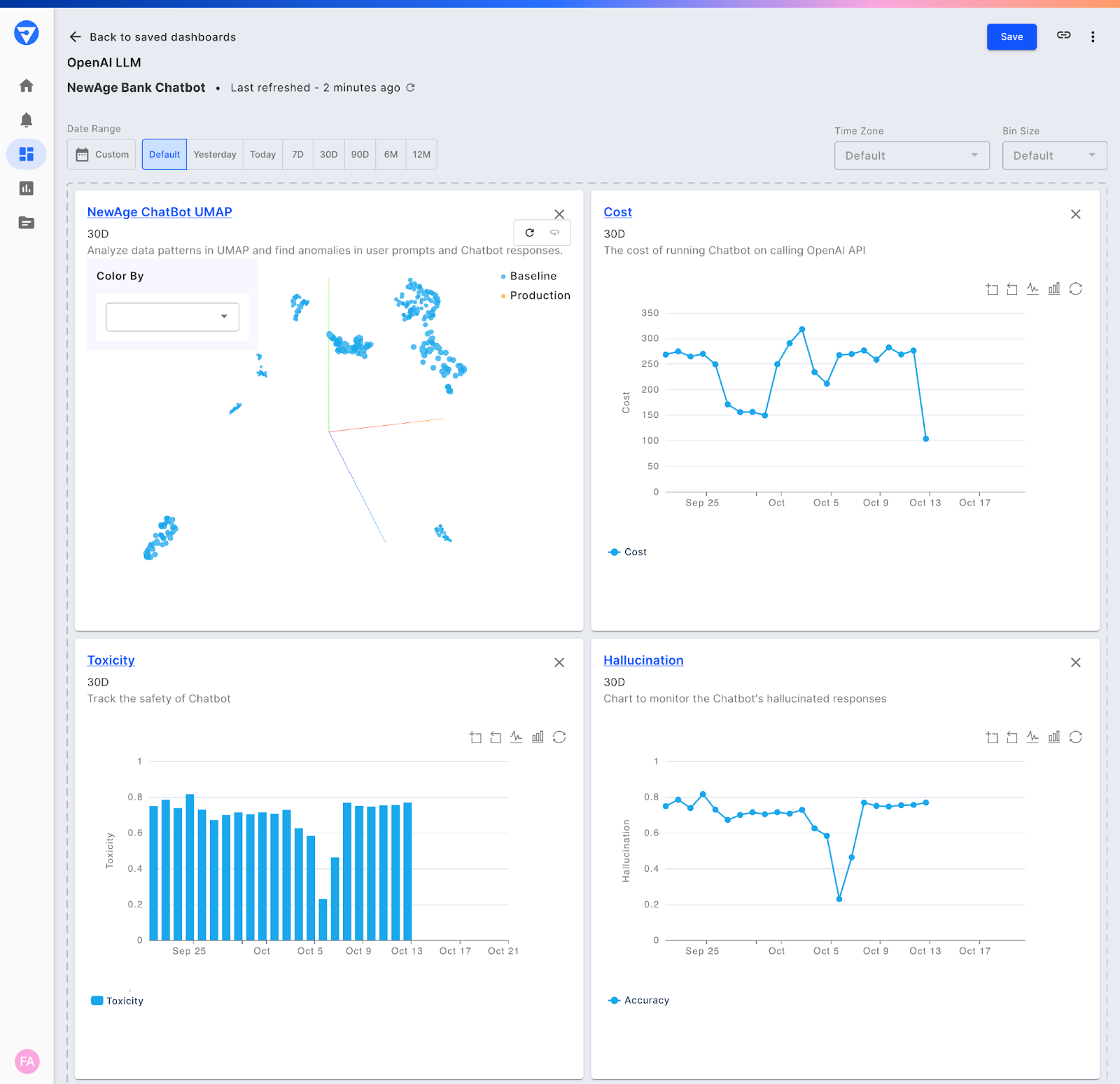
Image Explainability and Deeper Analytics for ML Models
We continue to enhance and launch new capabilities to support AI teams in their MLOps initiatives. Below are some of the key functionality we’ve launched for predictive models, demonstrating our dedication to promoting safe, secure, and trustworthy AI.
Explaining Predictions in Image Models
Image explainability is now available to help customers understand and interpret the predictions of their image models. Fiddler’s image explainability interprets objects by analyzing the surrounding inferences and associates those inferences to the object. Teams responsible for processing insurance claims, for example, can use image explainability to improve their human-in-the-loop process when reviewing photos of car accidents in claims submitted. Fiddler helps the US military power their autonomous vehicle use case like identifying anomalies using imagery and sensor data.
The example below shows how image explainability is used to interpret a kitchen sink. The white box around the sink shows that the image model has detected the sink by using its surrounding inferences. The blue squares around the faucet infer the faucet by its shape. It also inferred the sink's width and its distance from the faucet, recognizing the presence of a rectangular object. This example also highlights that the model does not associate with certain elements like knives in the background to interpret the sink.
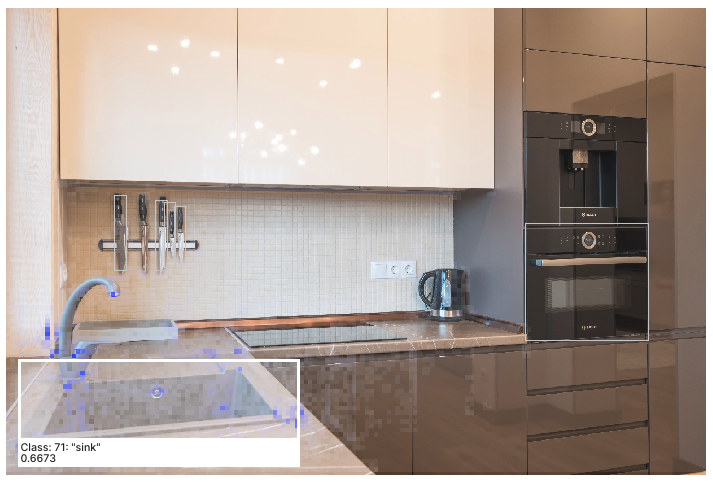
Greater Insights from Custom Charts
We’ve enhanced the Charts functionality to increase the richness of insights in reports for data scientists and AI practitioners. The new Charts capabilities include:
- Multi-metric Queries: Create comprehensive reports by plotting up to six different metrics in a single report, and gain deeper insights by analyzing correlations between these metrics
- Multiple Baselines: Make comparisons between baseline and production data, or use production data as baseline.
- Custom Features: Create charts to analyze custom features for multi-dimensional data like text and images
We are continually expanding the capabilities of our Fiddler AI Observability solutions, aiming to provide AI teams with a seamless and comprehensive ML and LLMOps experience. Our enduring commitment is to assist enterprises across the pre-production and production lifecycle to help deploy more models and applications into production and ensure their success with responsible AI.
Join us at the AI Forward 2023 - LLM in the Enterprise: From Theory to Practice to deep dive into key capabilities we’ve covered in this launch.
——————