What’s that Buzz?
If you haven’t heard about ChatGPT, you must be hiding under a very large rock. The viral chatbot, used for natural language processing tasks like text generation, is hitting the news everywhere. OpenAI, the company behind it, was recently in talks to get a valuation of $29 billion¹ and Microsoft may soon invest another $10 billion².
ChatGPT is an autoregressive language model that uses deep learning to produce text. It has amazed users by its detailed answers across a variety of domains. Its answers are so convincing that it can be difficult to tell whether or not they were written by a human. Built on OpenAI’s GPT-3 family of large language models (LLMs), ChatGPT was launched on November 30, 2022. It is one of the largest LLMs and can write eloquent essays and poems, produce usable code, and generate charts and websites from text description, all with limited to no supervision. ChatGPT’s answers are so good, it is showing itself to be a potential rival to the ubiquitous Google search engine³.
Large language models are … well… large. They are trained on enormous amounts of text data which can be on the order of petabytes and have billions of parameters. The resulting multi-layer neural networks are often several terabytes in size. The hype and media attention surrounding ChatGPT and other LLMs is understandable — they are indeed remarkable developments of human ingenuity, sometimes surprising the developers of these models with emergent behaviors. For example, GPT-3’s answers are improved by using the certain ‘magic’ phrases like “Let’s think step by step” at the beginning of a prompt⁴. These emergent behaviors point to their model’s incredible complexity combined with a current lack of explainability, have even made developers ponder whether the models are sentient⁵.
The Spectre of Large Language Models
With all the positive buzz and hype, there has been a smaller, forceful chorus of warnings from those within the responsible AI community. Notably in 2021, Timit Gebru, a prominent researcher working on responsible AI, published a paper⁶ that warned of the many ethical issues related to LLMs which led to her be fired from Google. These warnings span a wide range of issues⁷: lack of interpretability, plagiarism, privacy, bias, model robustness, and their environmental impact. Let’s dive a little into each of these topics.
Trust and Lack of Interpretability:
Deep learning models, and LLMs in particular, have become so large and opaque that even the model developers are often unable to understand why their models are making certain predictions. This lack of interpretability is a significant concern, especially in settings where users would like to know why and how a model generated a particular output.
In a lighter vein, our CEO, Krishna Gade, used ChatGPT to create a poem⁸ on explainable AI in the style of John Keats, and, frankly, I think it turned out pretty well.
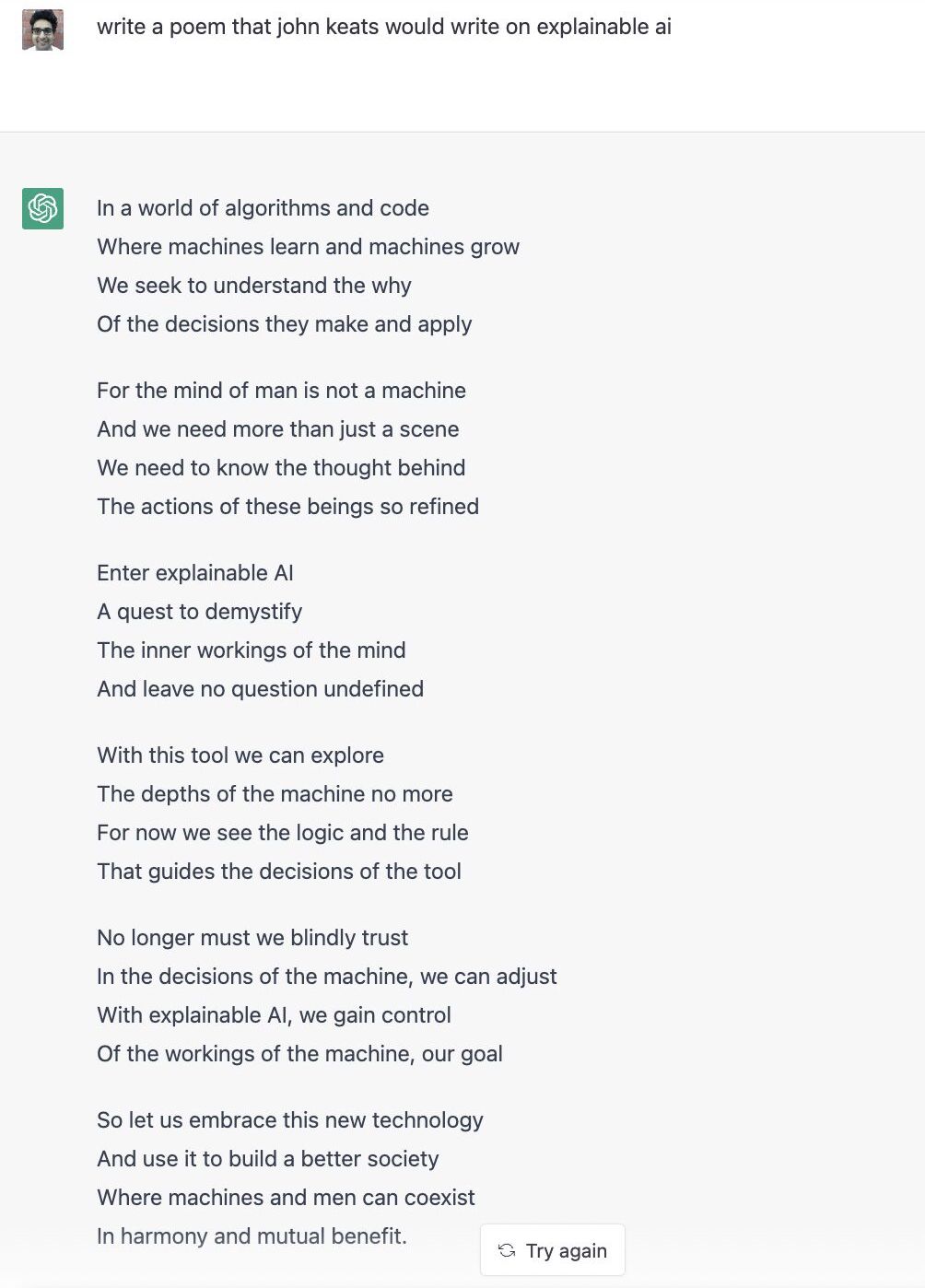
Krishna rightfully pointed out that the transparency around how the model arrived at this output is lacking. For pieces of work produced by LLMs, the lack of transparency around which sources of data the output is drawing on means that the answers provided by ChatGPT are impossible to properly cite and therefore impossible for users to validate or trust its output9. This has led to bans of ChatGPT-created answers on forums like Stack Overflow¹⁰.
Transparency and an understanding of how a model arrived at its output becomes especially important when using something like OpenAI’s Embedding Model¹¹, which inherently contains a layer of obscurity, or in other cases where models are used for high-stakes decisions. For example, if someone were to use ChatGPT to get first aid instructions, users need to know the response is reliable, accurate, and derived from trustworthy sources. While various post-hoc methods to explain a model’s choices exist, these explanations are often overlooked when a model is deployed.
The ramifications of such a lack of transparency and trustworthiness are particularly troubling in the era of fake news and misinformation, where LLMs could be fine-tuned to spread misinformation and threaten political stability. While Open AI is working on various approaches to identify its model’s output and plans to embed cryptographic tags to watermark the outputs¹², these solutions can’t come fast enough and may be insufficient.
This leads to issues around…
Plagiarism:
Difficulty in tracing the origin of a perfectly crafted ChatGPT essay naturally leads to conversations on plagiarism. But is this really a problem? This author does not think so. Before the arrival of ChatGPT, students already had access to services that would write essays for them¹³, and there has always been a small percentage of students who are determined to cheat. But hand-wringing over ChatGPT’s ability to turn all of our children into mindless, plagiarizing cheats has been on the top of many educators' minds and has led some school districts to ban the use of ChatGPT¹⁴.
Conversations on the possibility of plagiarism detract from the larger and more important ethical issues related to LLMs. Given that there has been so much buzz on this topic, I’d be remiss to not mention it.
Privacy:
Large language models are at risk for data privacy breaches if they are used to handle sensitive data. Training sets are drawn from a range of data, at times including personally identifiable information¹⁵ — names, email addresses¹⁶, phone numbers, addresses, medical information – and therefore, may be in the model’s output. While this is an issue with any model trained on sensitive data, given how large training sets are for LLMs, this problem could impact many people.
Baked in Bias:
As previously mentioned, these models are trained on huge corpuses of data. When data training sets are so large, they become very difficult to audit and are therefore inherently risky5. This data contains societal and historical biases¹⁷ and thus any model trained on it is likely to reproduce these biases if safeguards are not put in place. Many popular language models were found to contain biases which can result in increases in the dissemination of prejudiced ideas and perpetuate harm against certain groups. GPT-3 has been shown to exhibit common gender stereotypes¹⁸, associating women with family and appearance and describing them as less powerful than male characters. Sadly, it also associates Muslims with violence¹⁹, where two-thirds of responses to a prompt containing the word “Muslim” contained references to violence. It is likely that even more biased associations exist and have yet to be uncovered.
Notably, Microsoft's chatbot quickly became a parrot of the worst internet trolls in 2016²⁰, spewing racist, sexist, and other abusive language. While ChatGPT has a filter to attempt to avoid the worst of this kind of language, it may not be foolproof. OpenAI pays for human labelers to flag the most abusive and disturbing pieces of data, but the company they contract with has faced criticism for only paying their workers $2 per day and the workers report suffering from deep psychological harm²¹.
Model Robustness and Security:
Since LLMs come pre-trained and are subsequently fine tuned to specific tasks, they create a number of issues and security risks. Notably, LLMs lack the ability to provide uncertainty estimates²². Without knowing the degree of confidence (or uncertainty) of the model, it’s difficult for us to decide when to trust the model’s output and when to take it with a grain of salt²³. This affects their ability to perform well when fine-tuned to new tasks and to avoid overfitting. Interpretable uncertainty estimates have the potential to improve the robustness of model predictions.
Model security is a looming issue due to an LLM’s parent model’s generality before the fine tuning step. Subsequently, a model may become a single point of failure and a prime target for attacks that will affect any applications derived from the model of origin. Additionally, with the lack of supervised training, LLMs can be vulnerable to data poisoning²⁵ which could lead to the injection of hateful speech to target a specific company, group or individual.
LLM’s training corpuses are created by crawling the internet for a variety of language and subject sources, however they are only a reflection of the people who are most likely to have access and frequently use the internet. Therefore, AI-generated language is homogenized and often reflects the practices of the wealthiest communities and countries⁶. LLMs applied to languages not in the training data are more likely to fail and more research is needed on addressing issues around out-of-distribution data.
Environmental Impact and Sustainability:
A 2019 paper by Strubell and collaborators outlined the enormous carbon footprint of the training lifecycle of an LLM24,26, where training a neural architecture search based model with 213 million parameters was estimated to produce more than five times the lifetime carbon emissions from the average car. Remembering that GPT-3 has 175 billion parameters, and the next generation GPT-4 is rumored to have 100 trillion parameters, this is an important aspect in a world that is facing the increasing horrors and devastation of a changing climate.
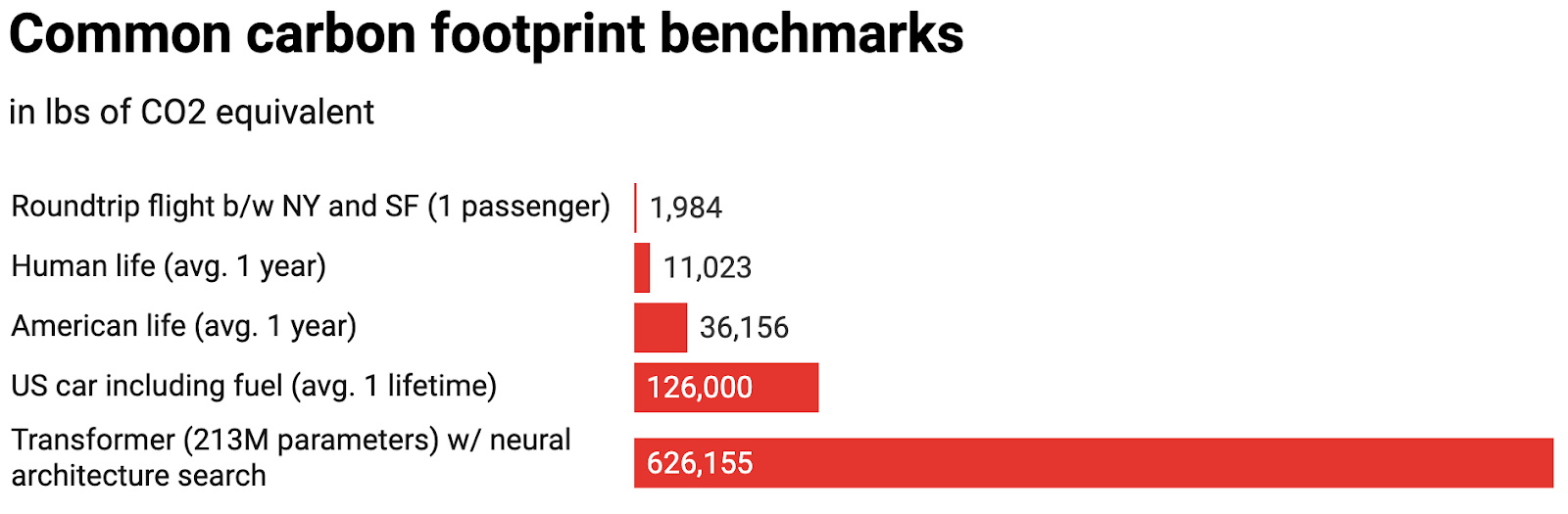
What Now?
Any new technology will bring advantages and disadvantages. I have given an overview of many of the issues related to LLMs, but I want to stress that I am also excited by the new possibilities and the promise these models hold for each of us. It is society's responsibility to put in the proper safeguards and use this new tech wisely. Any model used on the public or let into the public domain needs to be monitored, explained, and regularly audited for model bias. In part 2 of this blog series, I will outline recommendations for AI/ML practitioners, enterprises, and government agencies on how to address some of the issues particular to LLMs.
——
References:
- ChatGPT Creator Is Talking to Investors About Selling Shares at $29 Billion Valuation, WSJ, 2023.
- Todd Bishop, Microsoft looks to tighten ties with OpenAI through potential $10B investment and new integrations, GeekWire, 2023.
- Parmy Olson, ChatGPT Should Worry Google and Alphabet. Why Search When You Can Ask AI?, Bloomberg, 2022.
- Subbarao Kambhampati, Changing the Nature of AI Research, Communications of the ACM, 2022.
- Roger Montti, What Is Google LaMDA & Why Did Someone Believe It’s Sentient?, Search Engine Journal, 2022.
- Emily M. Bender, Timnit Gebru, Angelina McMillan-Major, Shmargaret Shmitchell, On the Dangers of Stochastic Parrots: Can Language Models be Too Big? FAccT 2021.
- Timnit Gebru, Effective Altruism Is Pushing a Dangerous Brand of ‘AI Safety’, Wired, 2022.
- Krishna Gade, https://www.linkedin.com/feed/update/urn:li:activity:7005573991251804160/
- Chirag Shah, Emily Bender, Situating Search, CHIIR 2022.
- Why posting GPT and ChatGPT generated answers is not currently acceptable, Stack Overflow, 2022.
- https://openai.com/blog/new-and-improved-embedding-model/
- Kyle Wiggers, OpenAI’s attempts to watermark AI text hit limits, TechCrunch, 2022.
- 7 Best College Essay Writing Services: Reviews & Rankings, GlobeNewswire, 2021.
- Kalhan Rosenblatt, ChatGPT banned from New York City public schools’ devices and networks, NBC News, 2023.
- Nicholas Carlini, Florian Tramer, Eric Wallace, Matthew Jagielski, Ariel Herbert-Voss, Katherine Lee, Adam Roberts, Tom Brown, Dawn Song, Ulfar Erlingsson, Alina Oprea, Colin Raffel, Extracting Training Data from Large Language Models, USENIX Security Symposium, 2021.
- Martin Anderson, Retrieving Real-World Email Addresses From Pretrained Natural Language Models, Unite.AI, 2022.
- Mary Reagan, Understanding Bias and Fairness in AI Systems, Fiddler AI Blog, 2021.
- Li Lucy, David Bamman, Gender and Representation Bias in GPT-3 Generated Stories, ACL Workshop on Narrative Understanding, 2021.
- Andrew Myers, Rooting Out Anti-Muslim Bias in Popular Language Model GPT-3, Stanford HAI News, 2021.
- James Vincent, Twitter taught Microsoft’s AI chatbot to be a racist asshole in less than a day, The Verge, 2016.
- Billy Perrigo, OpenAI Used Kenyan Workers on Less Than $2 Per Hour to Make ChatGPT Less Toxic, Time, 2023.
- Karthik Abinav Sankararaman, Sinong Wang, Han Fang, BayesFormer: Transformer with Uncertainty Estimation, arxiv, 2022.
- Andrew Ng, ChatGPT Mania!, Crypto Fiasco Defunds AI Safety, Alexa Tells Bedtime Stories, The Batch – Deeplearning.ai newsletter, 2022.
- Emma Strubell, Ananya Ganesh, Andrew McCallum, Energy and Policy Considerations for Deep Learning in NLP, ACL 2019.
- Eric Wallace, Tony Z. Zhao, Shi Feng, Sameer Singh, Concealed Data Poisoning Attacks on NLP Models, NAACL 2021.
- Karen Hao, Training a single AI model can emit as much carbon as five cars in their lifetimes, MIT Technology Review, 2019.
——
Originally published on Towards Data Science