The initial ChatGPT release caught everyone by surprise with its uncanny humanlike conversational abilities, quickly becoming the fastest adopted product in history. Simultaneously, its potential for misuse and possible unknown dangers has alarmed a lot of experts. Since its introduction, Machine Learning (ML) has always harbored hidden risks: model degradation risk, lack of decision transparency, bias etc. Large Language Models (LLMs) now add to that growing risk with safety, hallucination, and privacy risks.
With this backdrop, the EU introduced the EU AI Act as the world’s first comprehensive policy to regulate the use and development of AI to ensure the trust of EU citizens. The EU AI Act went into effect on August 1, 2024, with enforcement beginning on August 2, 2026. While European enterprises must comply with the EU AI Act by this enforcement date, other nations are expected to introduce more comprehensive AI regulations in the future. Enterprises outside of the EU are encouraged to thoroughly review the EU AI Act to anticipate upcoming regulations and prepare for responsible AI development and usage.
We explore the specifics of the EU AI Act and its implications for enterprises, with a particular focus on AI observability requirements, and discover how Fiddler can create a pathway to AI governance, risk management, and compliance (AI GRC).
Adapt and Comply to a Risk-Based AI Approach
The EU AI Act recommends a risk-based approach to classify AI applications:
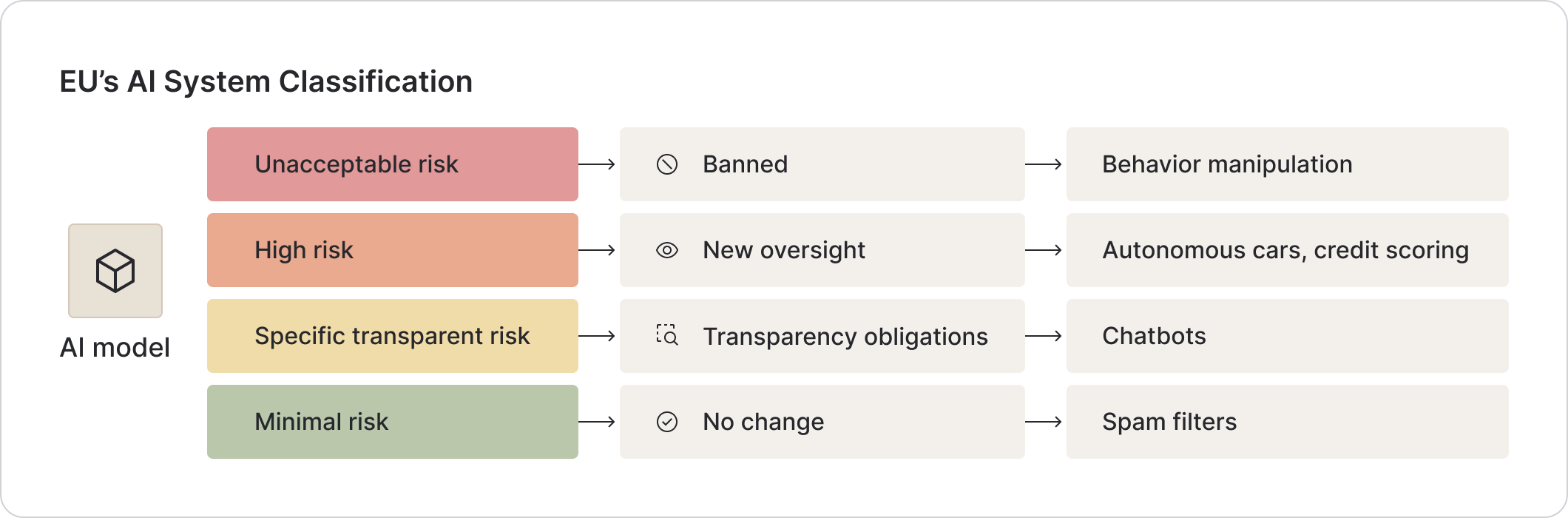
- Unacceptable risk applications include AI that threaten fundamental rights, such as dangerous toys, social scoring, certain predictive policing, and some biometric systems like workplace emotion recognition, are banned
- High risk applications will require rigorous AI compliance measures like pre-market assessments, regulatory audits, and EU database registration. Examples include biometrics, facial recognition, and AI in education, law enforcement, and healthcare like autonomous driving and credit scoring will have new oversight
- Specific transparent risk applications like chatbots and deepfakes must adhere to additional AI transparency rules, ensuring synthetic content is clearly marked and identifiable as artificially generated
- Other minimal risk applications like recommender systems and spam filters, which form a majority of AI applications, face no specific obligations but are encouraged to uphold trustworthiness and reliability
The AI Act: An AI Governance Framework Focused on High Risk Applications
The cornerstone of the EU AI Act is its guidelines for high-risk applications, which are classified as those that could endanger people (particularly EU citizens) and their opportunities, property, society, essential services, or fundamental rights. This classification includes applications such as recruitment, creditworthiness assessments, self-driving cars, and remote surgery. The Act also specifies that the extent of AI involvement in decision-making determines whether an application is classified as high-risk.
Consequences of Non-Compliance with the EU AI Act
AI compliance is required by the EU AI Act. Noncompliant companies face the following consequences:
- Financial Penalties: Fines of up to 7% of global annual turnover for serious violations, such as using banned AI applications
- Operational Disruptions: Non-compliant AI systems may be removed from the market, leading to significant revenue losses
- Reputational Damage: Failure to adhere to the Act's standards can erode consumer trust and tarnish a brand's image
- Increased Scrutiny: Risky AI deployments can lead to heightened legal and regulatory scrutiny, resulting in costly legal battles and diminished customer trust
- Market Access Limitations: Failure to meet AI compliance standards could restrict a company's ability to access the lucrative EU market, stifling growth opportunities and competitive edge
De-risk AI Applications with Fiddler AI Observability
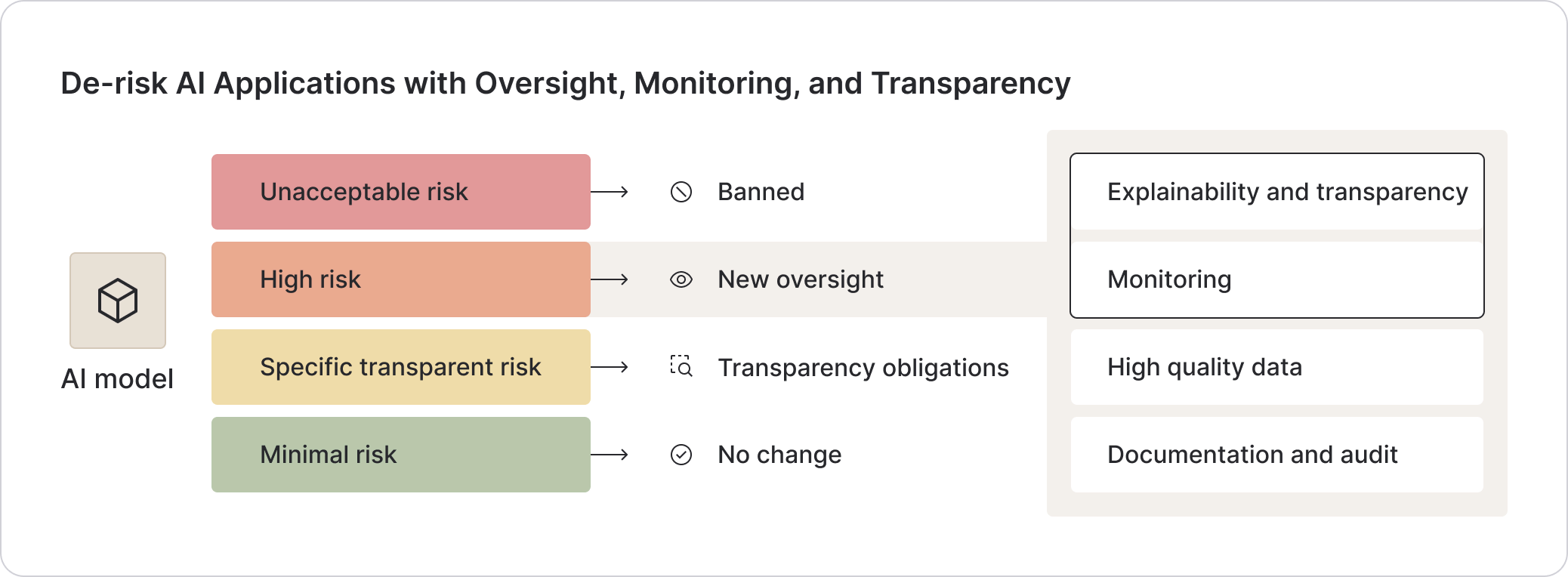
1. AI Transparency and Explainability
With the new AI Act, the EU is looking to address AI’s transparency problem. Most predictive models are an “opaque box” due to two traits of ML:
- Unlike other algorithmic and statistical models that are designed by humans, predictive models are trained on data automatically by algorithms.
- As a result of this automated generation, predictive models can absorb complex nonlinear interactions from the data that humans cannot otherwise discern.
This complexity obscures how a model converts input to output thereby causing a trust and transparency problem. Model complexity gets worse for modern deep learning models, making them even more difficult to explain and reason about.
Fiddler can help meet new AI compliance requirements with Explainable AI for ML that helps shine the light into the inner workings of AI models to ensure AI-driven decisions are transparent, accountable, and trustworthy. Explainable AI powers a deep understanding of model behavior to allow AI teams to debug and provide transparency around a wide range of models. LLMs are trained on such large datasets that explainability is still an unsolved research problem. However, there are newer approaches with Chain of Thought prompting and hallucination scores for LLM monitoring that provide reasoning, faithfulness, and groundedness context for deployed LLMs.
2. Monitoring with Human in the Loop
Predictive models are unique software entities, as compared to traditional code, in that they are probabilistic in nature. They are trained for high performance on repeatable tasks using historical examples. As a result, their performance can fluctuate and degrade over time due to changes in the model input after deployment. Depending on the impact of a high risk AI application, a shift in its predictive power could have a significant consequence on the use case, e.g. an ML model for recruiting that was trained on a high percentage of employed candidates will degrade if the real-world data starts to contain a high percentage of unemployed candidates, say in the aftermath of a recession. It can also lead to the model making biased decisions.
Monitoring these systems in a single pane of glass enables continuous operational visibility to ensure their behavior does not drift from the intention of the model developers and cause unintended consequences.
Generative AI models and applications can also be impacted by data drift, causing responses to vary over time for the same prompt. There are additional risks around safety, correctness, and privacy, as a result of hallucinations, jailbreak attempts or PII leakage.
Fiddler’s LLM Observability and ML Observability helps address all AI risks, including visibility, degradation, and other operational challenges for deployed models, ensuring AI compliance after the EU AI Act changes. The Fiddler AI Observability platform enables deployment teams to monitor model behavior, prevent drift, and mitigate unintended consequences, with alerting options to promptly address immediate operational issues.
3. Record Keeping
Since generative and predictive models, along with the data behind AI systems, are constantly evolving, continuous recording of model behavior is now essential for any operational use case. This involves logging all model inferences to enable future replay, inspection, root cause analysis, and explanation, facilitating auditing and remediation. The Fiddler AI Observability platform inherently provides an audit trail recording of all model logs for the effective monitoring and meeting of AI governance, risk, compliance requirements (AI GRC).
How to Stay Prepared for Upcoming AI Regulations
As new AI compliance regulations continue to emerge, enterprises must proactively prepare. The EU AI Act provides oversight for high-risk applications and encourages similar guidelines for lower-risk applications. This consistency can streamline the AI development process, allowing teams to follow uniform AI governance frameworks for all models.
Teams deploying AI models should bolster AI development by updating their AI infrastructure, processes, and tools to build trust and transparency into their predictive and generative models. By adding transparency into model performance and behavior, enterprises can instill customer trust and be well-prepared for upcoming regulations.
Contact our Fiddler AI experts to get started with AI Observability today and stay ahead of future AI compliance and regulatory changes.