As organizations shift from experimenting to operationalizing AI, data science and MLOps teams must prioritize explainable AI to maintain a level of trust and transparency within their models.
But what is explainable AI? Why is it becoming customary in the industry? And how should data science and MLOps teams think about explainable AI within their broader machine learning strategy?
In this Q&A, Fiddler Chief Scientist Krishnaram Kenthapadi shares key takeaways about the importance of explainable AI and how it connects responsible AI systems and model performance management. He also highlights the operational advantages, as well as the ethical benefits, of committing to AI design with explainability in mind.
How do you define explainable AI? And what are the different roles explainable AI plays across the broader AI market?
Explainable AI is a set of techniques to improve outcomes for all, including the businesses that deploy AI algorithms and the consumers who are affected by them. It is an effective way to ensure AI solutions are transparent, accountable, responsible, and ethical. Explainability enables companies to address regulatory requirements on algorithmic transparency, oversight, and disclosure, and build responsible and ethical AI systems.
As new data points get integrated into existing models, algorithm performance is likely to degrade or shift, resulting in data drift. Explainable AI mitigates this risk by making it easy for ML teams to recognize when it’s happening so they can then fix any issues and refine their models. Explainable AI is especially important for complex algorithms such as neural networks where there are multiple inputs fed into an opaque box, with little insight into its inner workings.
Within the enterprise, explainable AI is all about algorithmic transparency. AI developers need to know if their models are performing as intended, which is only possible if it’s clear how AI models arrive at their conclusions. Companies that employ AI only stand to gain if their innovations offer consistent and understandable results that lead to value-creating activities.
On the consumer side, explainable AI can improve the customer experience by giving people more context about decisions that affect them. For example, social media companies can tell users why they are subject to certain types of content, like Facebook’s Why am I seeing this post? feature. In the lending world, explainable AI can enable banks to provide feedback to applicants who are denied loans. In healthcare, explainable AI can help physicians make better clinical decisions, so long as they trust the underlying model.
The applications for explainable AI are far and wide, but ultimately, explainable AI guides developers and organizations in their pursuit of responsible AI implementation.
How will enterprises implementing explainable AI practices thrive?
While no company may intentionally want its products and services to suffer from gender or racial discrimination, recent headlines about alleged bias in credit lending, hiring, and healthcare AI models demonstrate these risks, and teach us that companies should not only have the right intent, but also take proactive steps to measure and mitigate such model bias. Given the high stakes involved, it’s critical to ensure that the underlying machine learning models are making accurate predictions, are aware of shifts in the data, and are not unknowingly discriminating against minority groups through intersectional unfairness. The solution? Model Performance Management (MPM).
MPM tracks and monitors the performance of ML models through all stages of the model lifecycle - from model training and validation to deployment and analysis, allowing it to explain what factors led to a certain prediction to be made at a given time in the past.
Explainability within MPM allows humans to be an active part of the AI process, providing input where needed. This ensures the opportunity for human oversight to course-correct AI systems and guarantees better ML models are built through continuous feedback loops.
What would you say to someone hesitant to implement explainable AI into their enterprise?
Explainable AI provides much-needed insight into how AI operates at every stage of its development and deployment, allowing users to understand and validate the “why” and “how” behind their AI outcomes. Algorithms are growing more complicated every day and, as time goes on, it will only get harder to unwind what we’ve built and understand the inner workings of our AI applications.
Implementing explainable AI is paramount for organizations that want to use AI responsibly. We need to know how our ML models reach their conclusions so that we can validate, refine, and improve them for the benefit of organizations and all citizens. It’s the crucial ingredient in a socially and ethically sound AI strategy. Explainable AI can help rebuild trust with skeptical consumers, improve enterprise performance, and increase bottom-line results.
How do you see the future of explainable AI evolving?
Explainable AI is becoming more important in the business landscape at large and is creating problems for companies that don’t have transparent ML models today. Therefore, much of the future of explainable AI will revolve around tools that support the end-to-end MLOps lifecycle.
MPM solutions that deliver out-of-the-box explainability, real-time model monitoring, rich analytics and fairness capabilities will help data science and MLOps teams build strong practices.
This support infrastructure for explainable AI is absolutely necessary given that nations worldwide are starting to implement AI regulations and take digital consumer rights more seriously. The EU’s recent Digital Services Act (DSA) provided a legal framework for protecting users’ rights across all online mediums, from social networks to mobile applications. The U.S. is contemplating an AI Bill of Rights that would accomplish a similar goal. In a world with more AI regulatory oversight, explainable AI, plus the tools that enable it, will be essential.
Learn more about explainable AI with our technical brief.
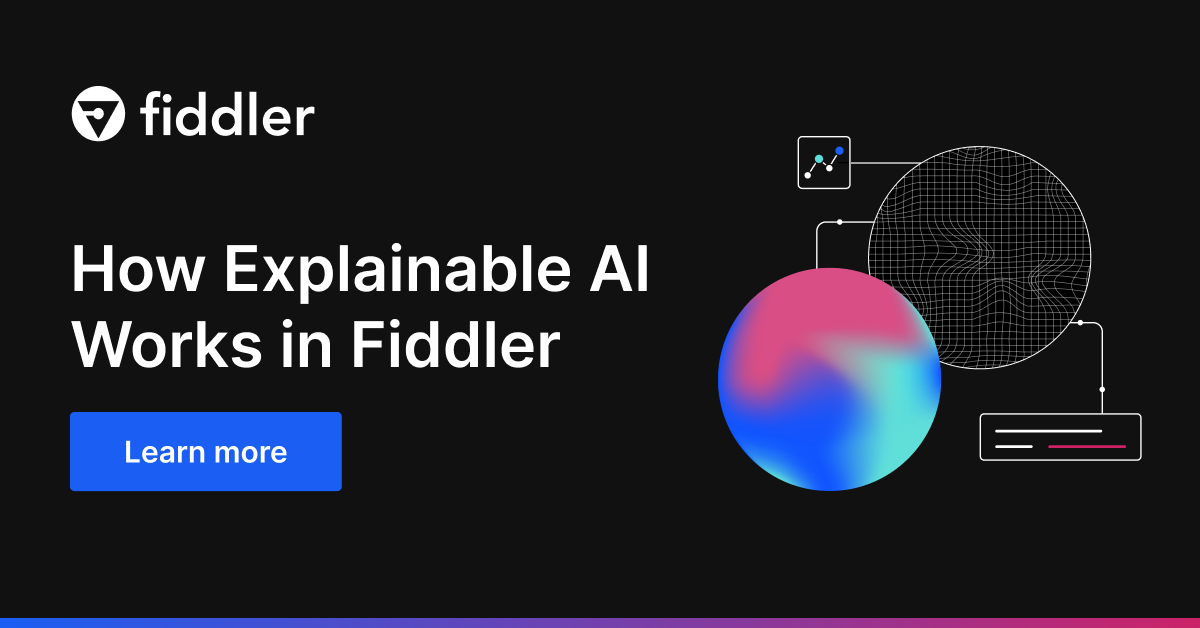