March is Women’s History Month, dedicated to celebrating the vital role of women in shaping world history and women’s contributions to areas like art, politics, culture, and science. We’ve been fortunate to speak with many incredible women who are at the forefront of machine learning research and applications, leading the way to develop new methodologies and responsible, ethical practices for working with AI systems. Today we’re featuring these women who are making history by building AI that works for everyone. Thank you for sharing your insights with all of us at Fiddler and in the broader community.
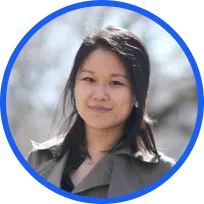
Karen Hao, Senior AI Reporter, MIT Technology Review
“With the recent AI revolution, I think there has always been this inherent belief that like any software, AI is meant to be developed at scale—that’s part of the benefit, that you develop something that works really well in one place and you can rapidly propagate it to other contexts and locations. But what I’ve realized in my reporting is that that’s actually the wrong model. The way to actually develop AI responsibly while being sensitive to so many cultures and contexts is to be hyper-specific when you develop AI.”

Manasi Joshi, Director of Software Engineering, Google
“Organizationally, I feel, why do we talk about responsibility? It’s because it’s not only limited to machine learning researchers who are doing the research or developers who are developing algorithms and building systems. It also matters to indirect users who have the impact of the products that they are using, because ultimately they are really exposed to that treatment exhibited by the product.”

Maria Axente, Responsible AI Lead, PwC UK
“Responsible AI for us is about building and using AI that looks at embedding ethics, governance, and risk management into a holistic approach end-to-end. It’s not a one-off. You don’t do ethics as a tick-box once. It’s everyone’s responsibility to pay attention at each stage of these issues, so that by the time we get to an outcome, we are much closer to achieving an ethical outcome than doing it with the current operating modalities—which are not fit for AI.”
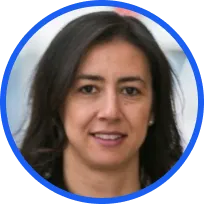
Merve Hickok, Founder, AIEthicist.org
“There are some great, encouraging examples of AI used for social good, and to create better opportunities and accessibility to resources for people. However, there are a couple of things that do worry me. The top one is the lack of any regulation and accountability on AI, especially in the US. You’re talking about a system having a potentially adverse impact on social justice and protection of privacy. I think we are past the point of birthing pains with AI and should kind of start focusing on how to grow a healthy child.”
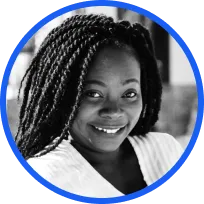
Michelle Allade, Director, Model Risk Management, MetaBank
“The model risk management function came from the banking industry and slowly got adopted into the insurance industry, but with the proliferation of AI and machine learning models across pretty much all different sectors, we can now see positions such as AI Risk Manager. And for my part I think it’s the right move, because anywhere models are being used, there’s definitely a need to have a risk management function.”

Narine Kokhlikyan, Research Scientist, Facebook
“We have more and more ML practitioners using ML for various different applications. And I think one thing that those model developers realize is that although they understand the theory, it’s not sufficient to actually say how does my model make decisions and how can we potentially influence those decisions. So I think that moving forward, the model developers, the ones who put the architecture together, they will put more emphasis on inherently interpretable models.”

Natalia Burina, AI Product Leader, Facebook
“A lot of this is new. So just thinking about it and having a plan in place and having a process, is industry-wide something that we haven’t been thinking about as much as we should. There’s this saying that planning is indispensable, plans are useless. I would just encourage everyone to push for a culture where we have a plan around responsible AI, because unless we have one, there’s not much that’s going to change.”

Sarah Bird, AI Product Leader, Microsoft
“One of the biggest misconceptions that we see in practice is that responsible AI can be ‘solved,’ that there’s something you do and then you’re just done—OK, we’ve implemented responsible AI and now we move on. It’s so much more like security, where there’s always going to be new things, there’s always going to be more you need to do. We need to recognize that this is a new practice. This is a new approach that we’re adding, and we’re never going to be done doing this.”

Sara Hooker, Research Scholar, Google Brain
“Feedback loops are starting to happen more frequently, where people are able to see algorithmic behavior, map it against their own experience, and articulate, ‘This isn’t what I expected, this doesn’t seem reasonable.’ And a good interpretability tool should make snafus less likely down the line when it’s too late to correct. It should allow people along the way to have the same degree of intuition to be able to audit. And that I believe has to be centered on showing subsets of the data that are most relevant for that user.”

Shalini Kantayya, Director, Coded Bias film
“I believe that we have a moonshot moment to make social change around ethics and AI. I really think that there is this relationship between the human imagination and what we actually create. And what I hope is that we can challenge technology even further, and imagine it to have some safeguards for democracy, invasive surveillance—just some guardrails in place to make sure that we use this powerful tool very responsibly.”

Tulsee Doshi, Fairness & Responsible AI Product Lead, Google
“There’s momentum globally to think about what it looks like for us to regulate AI. There are some things that we’re hearing especially around explainability and interpretability, and so I think over the next five years we’re going to see more and more documentation come out, more and more proposed regulations come out, and that is going to lead to more in all of our industries around actually putting in processes around explainability and interpretability. And it’s probably going to lead to a shift in computer science education.”
And of course, we are very grateful for the valuable contributions from our very own #WomenofFiddler to build more accountable and trustworthy AI: Brittany Bradley, Marie Beyene, Léa Genuit, Le An Pham, Mary Reagan, and Seema Shet. Thank you all for all that you do!