Model Monitoring for Enterprises

Never Let Monitoring or Anomalies Slow You Down
Want to decrease time-to-production and increase the number of models you release? How about resolving ML model issues with speed so you can achieve faster time-to-market?
The centralized dashboard delivers deep insights into model behavior and uncovers data pipeline issues to save debugging time. The Fiddler AI Observability platform operates at enterprise scale so you can go to market faster by monitoring and validating models during pre-deployment phases and releasing them in production.
- Catch and fix model inference violations right when they happen.
- Detect outliers and quickly assess which ones are caused by specific model inputs.
- Pinpoint data drift and contributing features to know when and how to retrain models.
Boost ML Models with Integrated Monitoring
Adoption of an enterprise-scale solution saves you the ongoing costs associated with building and managing an in-house ML monitoring solution. More importantly, it saves engineering and data scientists’ time so they can focus on what they do best: building ML models.
Reducing errors saves money, and delivering high-performance models to customers increases satisfaction and referrals.
- Lower costs by reducing mean time for issue identification and resolution.
- Decrease the number of errors to save money and engineering time.
- Grow revenue by increasing the number of models put in production in less time.
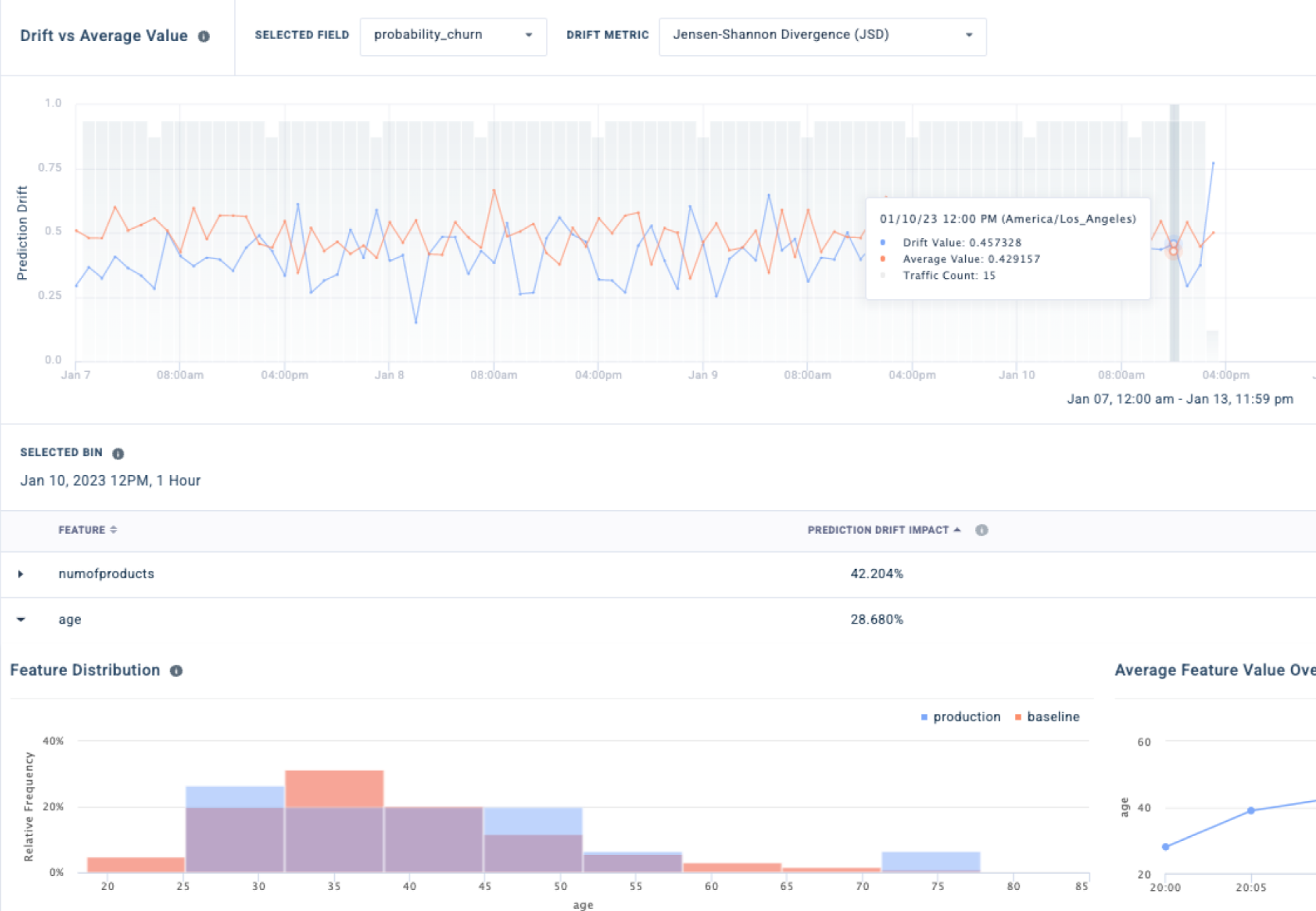

Leverage Teamwork and Talent with One-Stop Monitoring
If you have process silos and disparate monitoring solutions, you are at risk of operational inefficiencies, not to mention losing out on the benefits associated with collaboration.
Monitor all training and deployed models in one place for streamlined detection of data changes. The Fiddler intelligent platform empowers teams to come together, discover, discuss, and fix issues.
- Deliver a common platform with defined terminology to work across different MLOps teams.
- Enable multiple teams to work on and use a single model at the same time.
- Provide a unified dashboard with shared insights and custom real-time alerting.
- Optimize business outcomes by connecting model performance metrics to business KPIs.