Deliver Responsible AI
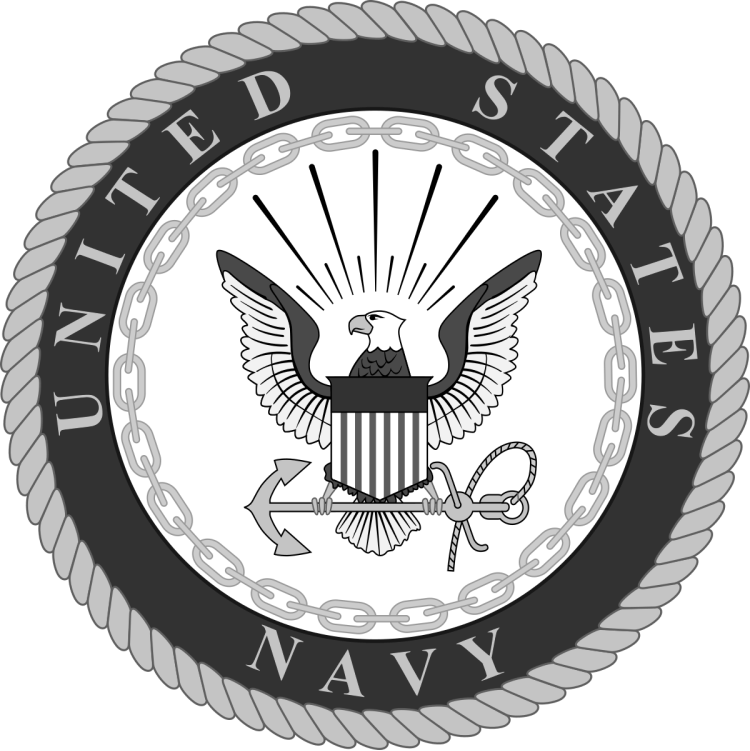
Shape a Culture of Accountability with Continuous Responsible AI
AI impacts lives. It’s more important than ever to build AI responsibly and adhering to this duty requires the detection and mitigation of bias, support for internal governance processes, and the reduction of risk through human involvement.
The Fiddler AI Observability platform brings ethics to the forefront. Through continuous real-time model monitoring, you ensure precise and rapid detection of bias in both datasets and ML models. With Fiddler, AI outcomes and predictions can be fair and inclusive.

Build and Deploy Responsible AI Solutions
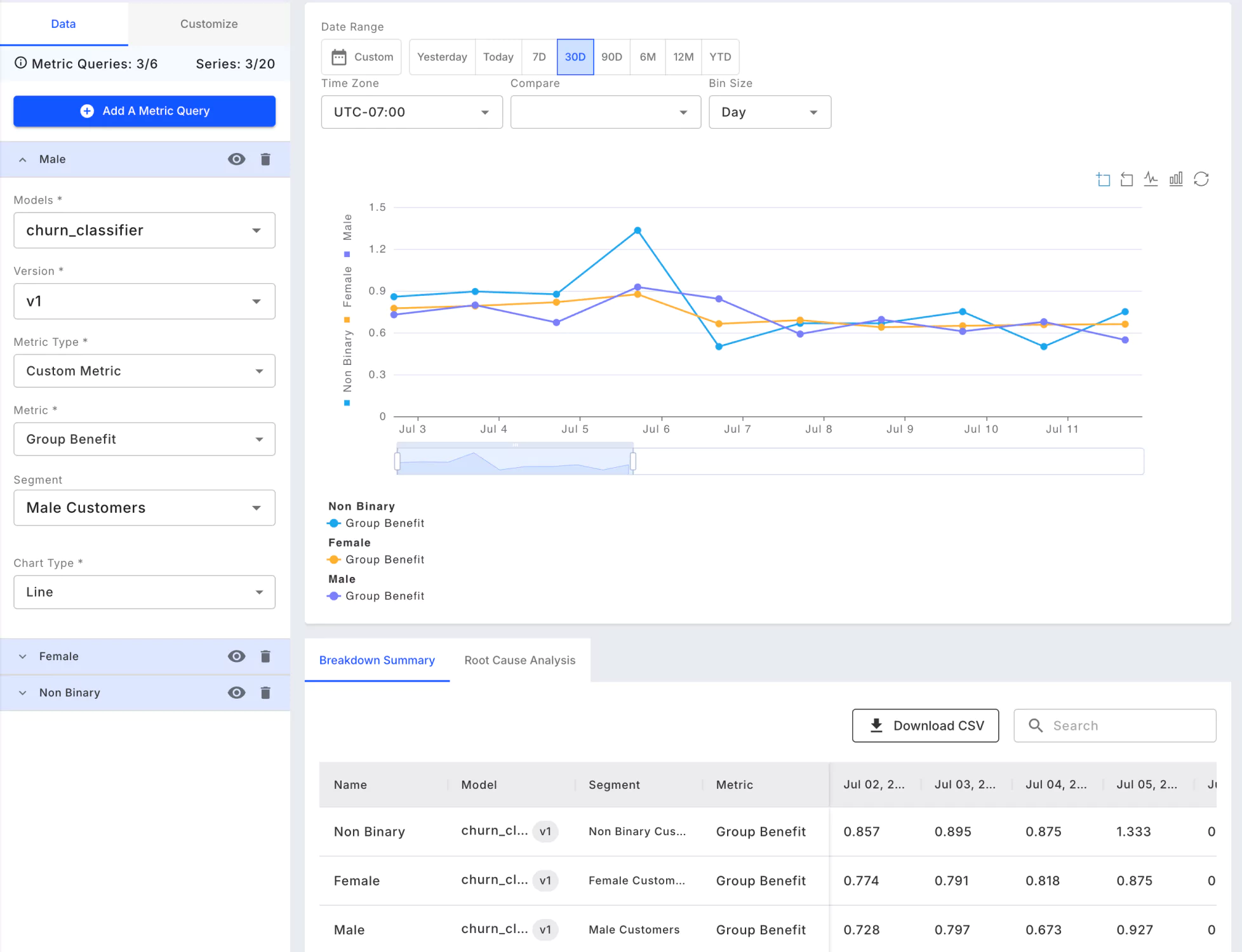
De-risk AI with Hidden Disparities
It’s almost impossible to ensure fairness in ML models if you don’t understand how models are behaving or why certain predictions are made. How can model bias be detected and assessed if you can’t extract causal drivers in your data and models?
Fiddler reduces model risk by enabling the deployment of AI governance and model risk management processes. Not only are coverage and efficiency increased, but human input into the decision-making loop for ML is enabled.
- Explain models in human-understandable terms to increase trust and transparency.
- Automate documentation of prediction explanations for governance requirements.
- Increase transparency and visibility into even the most complex models with explainable AI.
Develop Clear Guidelines for Fairness
No one wants to manage a PR catastrophe or incur fines and penalties.
Fiddler provides practical tools that support internal model governance processes and we provide practical tools, expert guidance, and white glove customer service to develop responsible AI practices.
Fiddler integrates deep explainable AI and analytics to help you grow into advanced capabilities over time and build a framework for responsible AI.
- Roll back models, data, and code to reproduce predictions and determine if bias was involved.
- Understand and explain decision-making factors to address customer complaints.
- Save money from fines and penalties by reducing occurrences.


Measure Fairness Metrics
How nice would it be to select multiple protected attributes at the same time to detect hidden intersectional unfairness? Or to benefit from fairness metrics when analyzing model performance?
With Fiddler, you can compare and measure a multitude of fairness metrics and evaluate, detect, and mitigate potential bias in both training and production datasets.
- Find deep-rooted biases with model performance metrics and analysis across protected classes.
- Deliver access to standard intersectional fairness metrics such as disparate impact, equal opportunity, and demographic parity.
- Provide model change and policy controls, coupled with analytics and reporting.