How to Track Fairness and Bias In Predictive and Generative AI
As predictive and generative AI (GenAI) models become more embedded in our daily applications and services, practicing responsible AI and implementing strong AI governance, risk, and compliance management (GRC) is crucial for oversight. A critical part of practicing responsible AI is ensuring fairness in both training data and model deployment so that all users, and organizations affected experience transparent, trustworthy, and equitable outcomes.
The Fiddler AI Observability platform enables enterprises to:
Monitor Fairness Metrics for Predictive and Generative Models
Fiddler empowers enterprises to track and visualize fairness and bias metrics for both predictive and generative AI models. In this blog, we'll explore key examples, including:
- For Predictive Models:
- Defining intersection of various protected attributes using segments
- Creating industry-specific metrics to track fairness and bias using custom metrics
- For Generative Models:
- Detect LLM responses that consist of racist or sexist content using the Fiddler Trust Service
Monitoring Fairness in Predictive Models
With Fiddler you can use model metadata and protected attributes from your datasets to track different aspects of fairness across the model lifecycle and on datasets the model is trained on.
The segments and metrics defined in the platform use the flexible Fiddler Query language (FQL) interface to allow your team to define Pythonic conditions and calculations via Fiddler’s UI or API.
Define Intersectionality Using Segments
Segments let you define the intersection of several protected attributes like race, gender, sexual orientation, and any other attributes to ensure your models are fair and not biased towards any specific group.


Define Industry-Specific Fairness Metrics using Custom Metrics
Custom metrics let you define metrics used in your industry to measure the fairness of outcomes for the decisions made by models used by your organization.

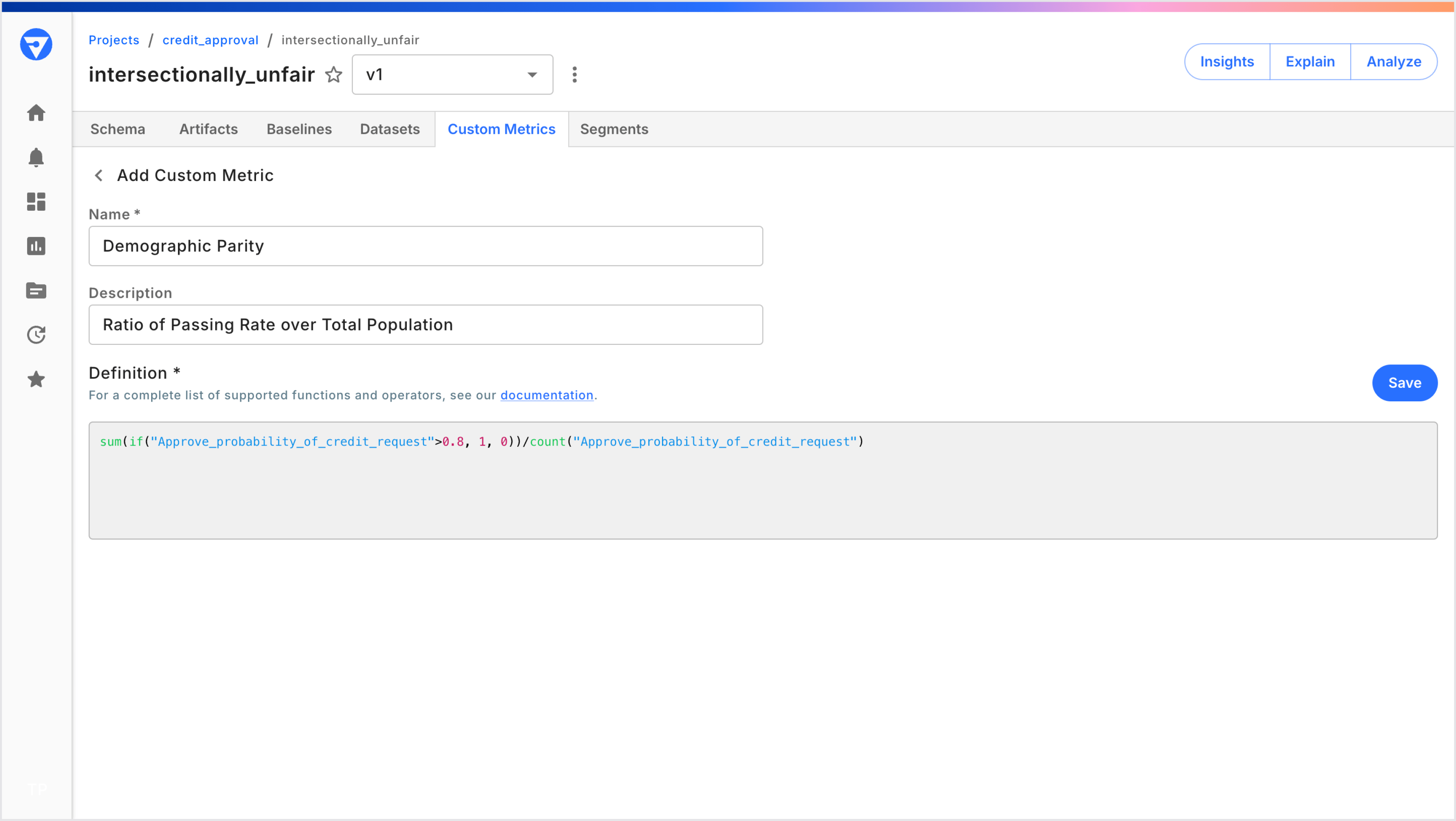
Monitoring Fairness in Generative AI Models
With the advent of LLMs, new challenges in maintaining fairness have surfaced. These models can unintentionally generate biased or harmful content, highlighting the need for proactive monitoring and prevention. Fiddler AI addresses this challenge with its Fiddler Trust Service, consisting of proprietary Fiddler Trust Models that are fast, scalable, secure, and cost-effective in monitoring.
Fiddler Trust Service helps enterprises with:

Similar to predictive models, custom metrics and segments can further stratify behavioral signals from safety models, allowing you to build targeted metrics and alerts that engage the appropriate teams effectively.
For example, the segment below tracks LLM responses containing biased language that could harm users or the business and generates alerts to notify a moderator when such instances are detected.

Customized Dashboards with Fairness Reports
Start tracking model behavior by incorporating fairness reports into dashboards customized for Trust and Safety, and Risk and Compliance teams. These dashboards and reports can also be shared for third party reviews and for GRC purposes.
When any of these metrics fall outside the enterprise's accepted thresholds, alerts notify the model development, engineering, or risk and compliance teams to intervene and identify the root cause of unfair model outcomes. This proactive approach helps address issues and tune the model to reduce the likelihood of bias in the future and remain compliant with GRC standards.


Monitoring Fairness Metrics is Essential for Responsible AI and GRC Compliance
Gaining oversight on AI bias and fairness metrics has become easier for enterprises deploying ML and GenAI models, thanks to the Fiddler AI Observability platform. By leveraging custom segments, custom metrics, and the Fiddler Trust Service for LLM monitoring, AI teams can proactively detect unfair outcomes, reduce AI risks, and remain compliant with GRC standards. Enterprises can avoid AI disasters caused by bias and unfair outcomes, ensuring their AI implementations are equitable and fair for all.
Connect with our Fiddler AI experts to learn how to integrate fairness metrics into your responsible AI framework and ensure GRC compliance.