Last week, the Explainable AI Summit, hosted by Fiddler Labs, returned to discuss top-of-mind issues that leaders face when implementing AI. Over eighty data scientists and business leaders joined us at Galvanize to hear from the keynote speaker and Fiddler’s head of data science, Ankur Taly, and our distinguished panelists moderated by our CEO Krishna Gade:
- Manasi Joshi, Engineering Director of ML Productivity, Google Brain
- Peter Skomoroch, Former Head of AI Automation, Workday
- Sarah Aerni, Director of Data Science and Engineering, Salesforce
- Yang Wong, Data Scientist, Affirm
- Naren Chittar, Executive Director, JPMorgan Chase

Summary
Reprising some topics from our summit in February, the H2 summit focused on explainability techniques and industry-specific challenges.
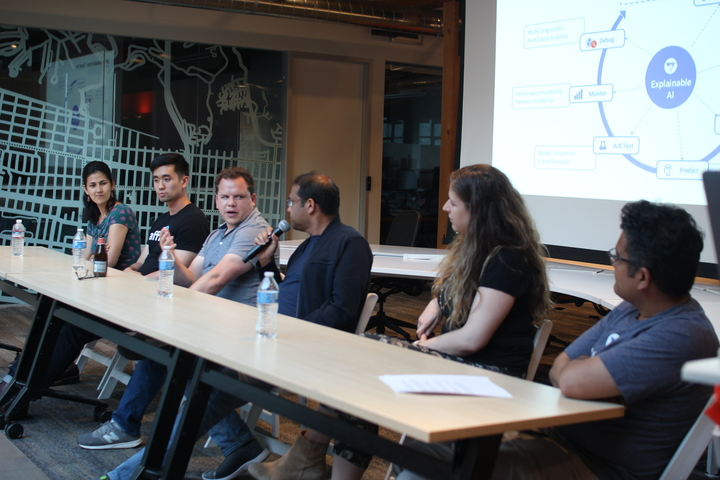
Takeaway #1: In financial services, companies are working through regulatory and technical hurdles to integrate machine learning techniques into their business model.
Financial services understand the potential of AI and want to adopt machine learning techniques. But they are reasonably wary of running afoul of regulations. If someone suspects a creditor has been discriminatory, the Federal Trade Commission explicitly suggests that he or she consider suing the creditor in federal district court.
Banks and insurance companies already subject models to strict, months-long validation by legal teams and risk teams. But if using opaque deep learning methods means forgoing certainty around model fairness, then these methods cannot be a priority.
However, some use cases are less regulated or not regulated at all, allowing financial services to explore AI integration selectively. Especially if regulators continue to accept that AI may never reach full explainability, AI usage in financial services will increase.

Takeaway #2: And across all industries, leaders are prioritizing trustworthiness of their models.
Most companies understand the risk to their brand and consumer trust if models go awry and make poor decisions. So leaders are implementing more checks before models are promoted to production.
Once in production, externally facing models generate questions and concerns from customers themselves. Business leaders are seeing the need to build explainability tools to address these questions surrounding content selection. Fortunately, many explainability tools are available in open source, like Google’s TCAV and Tensorflow Model Analyzer.
And as automated ML platforms attract hundreds of thousands of users, platform developers are ramping up education about incorrect usage. Ramping up education is necessary but not sufficient. ML Platforms should assist modelers with capabilities to inspect model behavior against sub groups of choice to inform if there is potential bias as manifested by their models.
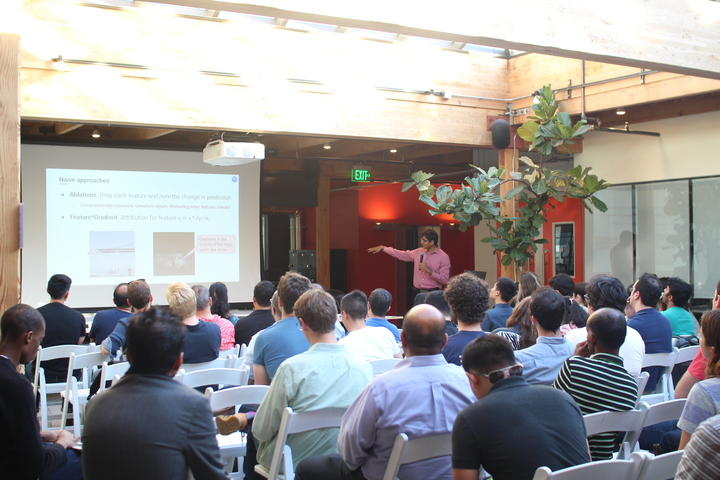
Takeaway #3: Integrated Gradients is a best-in-class technique to attribute a deep network’s (or any differentiable model’s) prediction to its input features.
A major component of explaining an AI model is the attribution problem. For any given prediction by a model, how can we attribute that prediction to the model’s features?
Currently, several approaches in use are ineffective. For example, an ablation study (dropping a feature and observing the change in prediction) is computationally expensive and misleading when features interact.
To define a better attribution approach, Ankur Taly and his co-creators first established the desirable criteria, or axioms. One axiom, for instance, is insensitivity: a variable that has no effect on the output should get no attribution. These axioms then uniquely define the Integrated Gradients method, which is described by the equation below.

Integrated gradients is easy to apply and widely applicable to differentiable models. Data science teams should consider this method to evaluate feature attribution inexpensively and accurately.
Acknowledgments
Thank you to Galvanize for hosting the event, to our panelists, and to our engaged audience! We look forward to our next in-person event, and in the meantime, stay tuned for our first webinar. For more information, please contact us.
